A team from UC San Francisco, including members of UCSF's Center for Intelligent Imaging (ci2), developed and tested a deep learning system to automate the identification of several findings on a lumbar spine Magnetic Resonance Imaging (MRI).
The researchers share their conclusions in "Deep learning for automated, interpretable classification of lumbar spinal stenosis and facet arthropathy from axial MRI," published in European Radiology. UCSF ci2's Upasana Upadhyay Bharadwaj, MBBS, a postdoctoral scholar at the UCSF Department of Radiology and Biomedical Imaging, is the first author of the paper. The senior author is Sharmila Majumdar, PhD, co-executive director of ci2.
The investigators found that the system performed well at automated interpretation of lumbar spine MRI including the classification of central canal stenosis, neural foraminal stenosis and facet arthropathy.
"While existing deep-learning systems are opaque, leading to clinical deployment challenges, the proposed system is accurate as well as interpretable, providing valuable information to a radiologist in clinical practice," write Bharadwaj et al.
The researchers used 200 axial MRI studies from 2008 to 2019 and grouped through studies into training, validation and testing subsets. The accuracy of the model was comparable to inter-reader agreement scores, showing the potential of these programs to assist in clinical practice.
Co-authors of the article include ci2's Valentina Pedoia, PhD, Thomas Link, MD, PhD, and Cynthia Chin, MD, UCSF Radiology's Miranda Christine and Steven Li, and Dean Chou, MD, of the UCSF Department of Neurological Surgery.
Read more about research and news at UCSF Ci2.
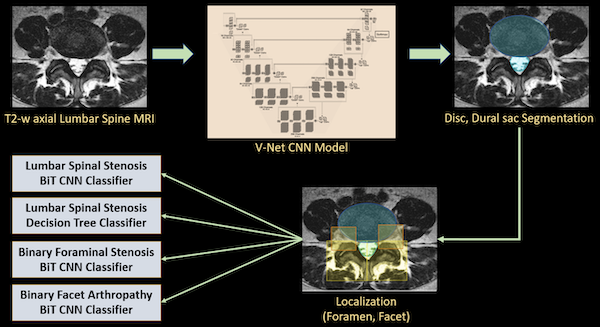