A research team, including senior author Yang Yang, PhD, from the UC San Francisco Department of Radiology and Biomedical Imaging, tested the utility of trained models to diagnose five types of interstitial lung disease (ILD) and determine a patient's 3-year survival rate.
The investigators share their conclusions in "Interstitial lung disease diagnosis and prognosis using an AI system integrating longitudinal data," published in Nature Communications.
The team used a RadImageNet-pretrained model to diagnose the type of ILD and a transformer model to estimate the survival rate. The models were provided initial chest computed tomography (CT) scans and clinical history.
The researchers found that their diagnostic joint deep convolutional neural network model "accurately predicted the five ILD subtypes and outperformed a senior thoracic radiologist and senior pulmonologist in diagnosing true cases of usual interstitial pneumonia (UIP)." The Transformer model was able to predict a patient's survival rate with high sensitivity.
"The proposed deep learning system demonstrates high potential in accurately diagnosing five subtypes of ILD," write the authors. "This could help clinicians without access to specialized thoracic training fellow to diagnose and make dynamic predictions regarding patient prognosis and disease progression."
ILD is a disease category that refers to more than 200 pulmonary conditions. Diagnosing and classifying the condition via CT can be straightforward, but imaging findings can also overlap or be difficult to identify, making interpretation challenging and dependent on the individual radiologist. The purpose of the study was to alleviate some of that difficulty by developing an AI system to:
- Classify five different types of ILD based on chest CT scans and clinical history
- Monitor patient disease progression.
The results of the study highlight the utility of this deep learning system to aid clinicians in diagnosis, classification, progression and prognosis of ILD.
"We believe the proposed models … demonstrate equivalent performance to a senior thoracic radiologist and a senior pulmonologist and also evaluate survival rate at each follow-up visit, which could be a useful tool to distinguish ILD subcategories and manage the long-term progression of patients," write Mei et al.
Dr. Yang is an associate professor in residence at the UC San Francisco Department of Radiology and Biomedical Imaging. The first author is Xueyan Mei of the Icahn School of Medicine at Mount Sinai. The other co-authors on the paper are faculty, students and trainees at Mount Sinai. The study involved data from the Mount Sinai Medical Center Research Registry for Interstitial Lung Disease (MSMC-ILD).
Read more about research and news at the UCSF Center for Intelligent Imaging (ci2).
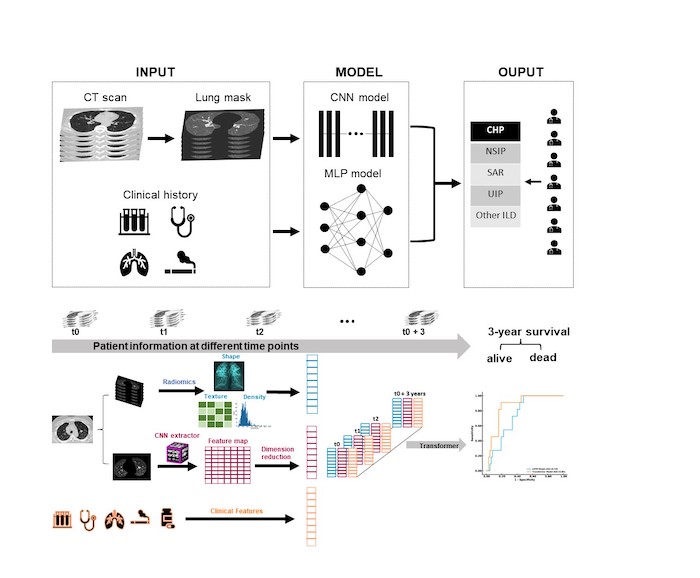