Evan Calabrese, MD, PhD, an assistant professor of radiology at Duke University Medical Center and a physician-scientist researcher at the Duke Center for Artificial Intelligence in Radiology (DAIR), recently presented at UCSF’s Center for Intelligent Imaging (ci2) SRG Pillar meeting. Dr. Calabrese pursued his radiology residency and neuroradiology fellowship at UCSF and continues to collaborate with UCSF on various projects. His presentation provided a detailed analysis of his research on artificial intelligence (AI) applications in pediatric neuroradiology, focusing on neonatal hypoxic-ischemic encephalopathy (HIE).
Despite his primary focus on neuro-oncology, Dr. Calebrese highlighted the underexplored potential of AI in pediatric neuroradiology, emphasizing AI's role in improving outcomes and prognostication in neonatal HIE, a condition with significant morbidity and mortality rates.
Neonatal HIE impacts neonatal mortality and long-term neurodevelopmental outcomes. Dr. Calabrese noted the importance of neonatal brain MRI within the first week of life for assessing suspected HIE but acknowledged the complexity and variability in interpreting these images. The challenge is accurately correlating neuroimaging results with long-term outcomes, crucial for patient care, resource allocation, and clinical trial enrollment.
Dr. Calabrese described how he uses the SMART goals framework to evaluate the feasibility of new AI projects. For the HIE project, the goal was to predict neurodevelopmental impairment at two years of age using neurodevelopmental testing metrics. The dataset from the HEAL trial included brain MRI data from 500 neonates across 17 sites. Despite its richness, it required meticulous manual review and curation due to variability in imaging protocols and data quality issues.
Initial analyses showed significant correlations between diffusion-weighted imaging (DWI) abnormalities and neurodevelopmental outcomes, particularly in the thalamus and perirolandic cortex. These findings provided a basis for developing the AI model, named AI OPiNE (Outcome Prediction in Neonatal Encephalopathy), which aimed to predict long-term neurodevelopmental outcomes following HIE by integrating raw imaging data, radiomic features, and clinical data. The model employed a deep convolutional neural network for image feature encoding and a multi-layer perceptron for classification, validated by holding out entire institutions as an out-of-sample test set.
AI OPINE demonstrated moderate predictive performance with an area under the receiver operating characteristic curve of 0.74, comparable to a scoring system by three expert pediatric neuroradiologists. This indicated AI's potential to match expert human performance in prognostication for neonatal HIE.
Dr. Calabrese also discussed feature fusion in AI models, critiquing early feature fusion for its susceptibility to modality dominance and exploring late feature fusion and decision fusion as potential solutions. He emphasized the need for empirical trials to determine the most effective approach.
Dr. Calabrese addressed the balance between bias and variance in classification models, illustrating this with a complexity-performance curve. Simpler models like decision trees risk underfitting, while complex models like artificial neural networks risk overfitting. The challenge lies in identifying the appropriate model complexity for specific tasks. He suggested experimenting with various complexities to achieve an optimal balance.
Output considerations were another crucial aspect. Dr. Calabrese highlighted the importance of stakeholder and ethical considerations in presenting model results, ensuring transparency, and including potential caveats and performance metrics across different patient subgroups. This emphasized the importance of collaboration and data sharing in AI research. He shared that he’s committed to creating and sharing open datasets, particularly for neonatal encephalopathy research, aiming for significant advancements in the field.
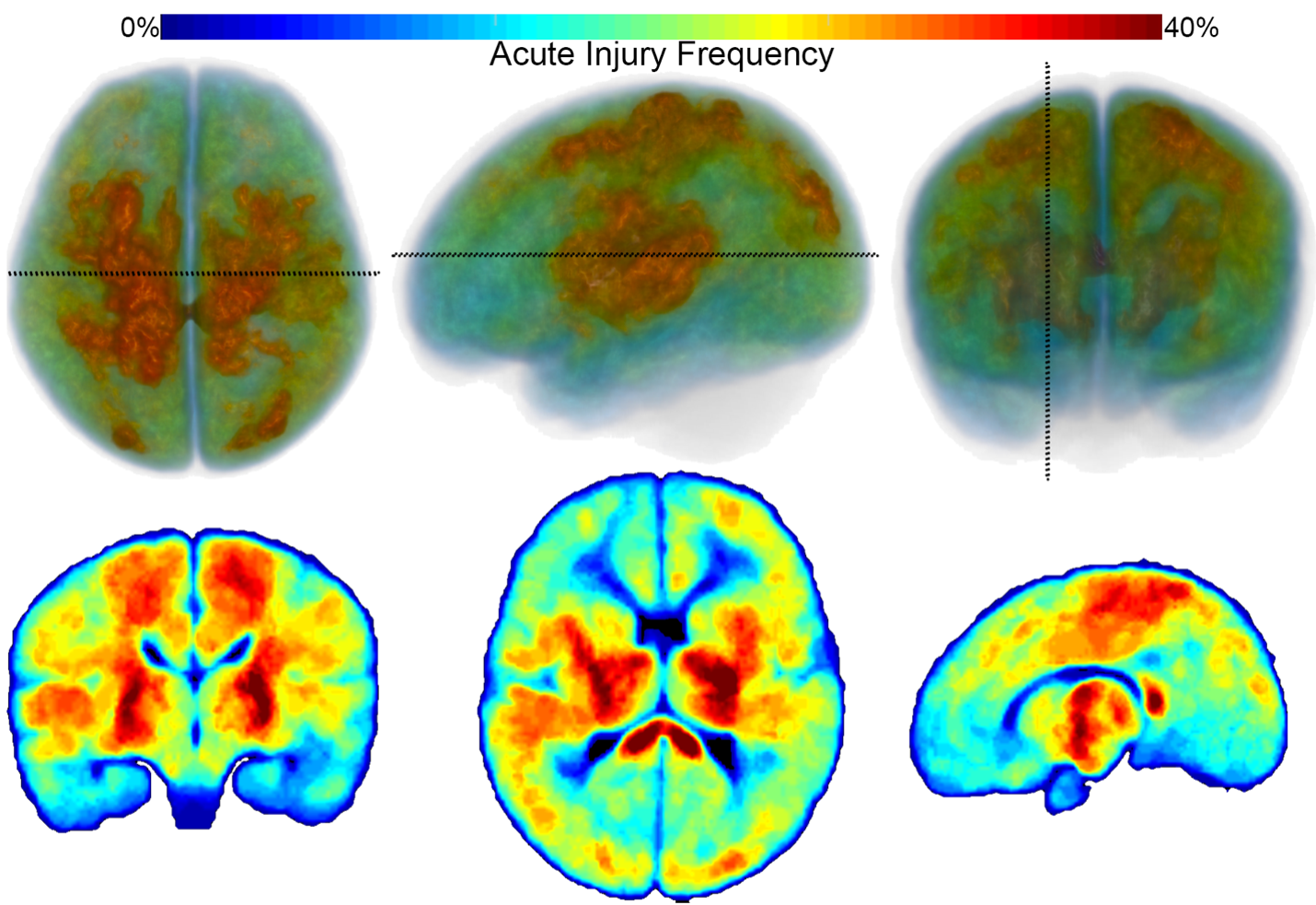