A large international research team, including investigators from UC San Francisco's Center for Intelligent Imaging (ci2), present a federated machine learning (FL) model that automatically generates a three-dimensional tumor boundary on MRI for patients with glioblastoma. FL allows multiple sites around the world to train a shared deep learning model without sharing any confidential patient image data.
The investigators share their conclusions in "Federated learning enables big data for rare cancer boundary detection." The research, which involves data from 71 sites across six continents, is the largest FL study to date. The work is published in Nature Communications.
UCSF ci2's Evan Calabrese, MD, PhD, and Javier Villanueva-Meyer, MD, and UCSF Department of Radiology and Biomedical Imaging's Soonmee Cha, MD, are among the co-authors of this paper, which was led by first author Sarthak Pati, MS, of the University of Pennsylvania.
The researchers found that the FL model had a 33% tumor boundary delineation improvement for the surgically targetable tumor, and a 23% improvement for the complete tumor extent, over a publicly trained model. The FL model showed the most significant improvement over the publicly trained model for the more challenging sub-compartments of boundary detection.
"This finding of FL benefiting the more challenging tasks rather than boosting performance on the relatively easier task by gaining access to larger amounts of good quality data holds a lot of promise for FL in healthcare," write Pati et al.
The study involved data from 6,314 glioblastoma patients, including nearly 400 contributed by UCSF researchers, and the authors noted that "it is the use of FL that successfully enable access to such an unprecedented dataset of the most common and fatal adult brain tumor." Because of the importance of glioblastoma boundary detection for clinical decision-making and treatment-planning, the FL model could have an important and vast impact.
In addition to sharing the findings on glioblastoma tumor boundary detection, the investigators highlighted the potential of FL to aid clinical work and relieve some of the workload and pressure on clinicians.
"Recent technological advancements in healthcare … have resulted in a radical growth of primary observations generated by health systems," write Pati et al. "This contributes to the burnout of clinical experts, as such observations require thorough assessment … Advances in [machine learning], and particularly deep learning (DL), have shown promise in addressing these complex healthcare problems."
The team anticipates that the study will:
- Enable more healthcare studies informed by large diverse data
- Facilitate further research into glioblastoma by releasing the team's consensus model
- Demonstrate the effectiveness of FL at a large scale
Dr. Calabrese was a resident and fellow at UCSF and is now Assistant Professor at Duke University. Dr. Villanueva-Meyer is an Associate Professor at UCSF Radiology. Dr. Cha is a Professor at UCSF Radiology and the Program Director of the diagnostic radiology residency program.
Read more about research and news at UCSF Ci2.
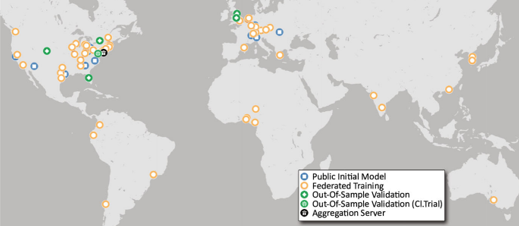
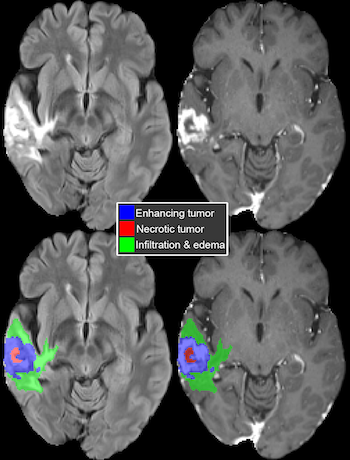