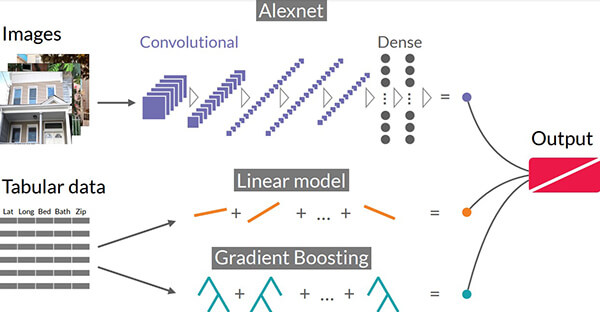
After three years, investigators from the UCSF Center for Intelligent Imaging (ci2) are very excited to announce that their work on Representational Gradient Boosting (RGB) has just been published! The team includes Gilmer Valdes, PhD, assistant professor at both UCSF Radiation Oncology and UCSF Epidemiology and Biostatisics; Stathis Gennatas, MBBS, PhD, assistant professor at UCSF Epidemiology & Biostatistics; Fei Jiang, PhD, MS, assistant professor at UCSF Epidemiology & Biostatistics and Jerome Friedman, Stanford University professor emeritus and one of the world's leading researchers in statistics and data mining.
Presently, artificial neural networks are the predominant class of algorithms in this area, known as representational learning. The team introduces RGB which is a nonparametric algorithm that estimates functions with multi-layer architectures obtained using backpropagation in the space of functions. Read more in IEEE Transactions on Pattern Analysis and Machine Intelligence (TPAMI), a flagship journal published by the IEEE Computer Society leading the field of artificial intelligence.
Why RGB? "Medical datasets are normally multimodal in nature (i.e., images e.g., CT, MRI and tabular data e.g., comorbidities and lab values). Usually, it is the case that the best function to analyze each modality is different (e.g CNN for images and Gradient Boosting for Lab values)," says Dr. Valdes. "RBG establishes the theory to train these functions at the same time. As such, it enables investigators to integrate complex medical data into the same optimization regime to enable precision medicine."
Great work by this collaborative, multidisciplinary team. We look forward to seeing what's next!