A team of researchers from UC San Francisco's Department of Radiology and Biomedical Imaging and Center for Intelligent Imaging (ci2) tested a deep-learning method for knee magnetic resonance imaging (MRI).
The researchers share their conclusions in "Synthetic Knee MRI T1p Maps as an Avenue for Clinical Translation of Quantitative Osteoarthritis Biomarkers," published in MDPI Bioengeering. Michelle W. Tong, the lead author, is a graduate student in the joint University of California, Berkeley - University of California San Francisco bioengineering program and ci2 member. Colleagues from UCSF's Department of Radiology and Biomedical Imaging also contributed to the research.
The researchers trained a 2D U-Net to generate synthetic T1p maps from T2 maps for knee MRI to facilitate clinical translations of quantitative osteoarthritis biomarkers. "The deep neural network synthesized high-fidelity reconstructions of T1p maps, preserving textures and local T1p elevation patterns in cartilage," writes Dr. Tong and co-authors.
The investigators used a dataset of 509 healthy contralateral and injured ipsilateral knee images from patients with ACL injuries and reconstruction surgeries. Analysis of the T1p maps within cartilage compartments "revealed minimal bias ( 0.10 ms), tight limits of agreement, and quantification error (5.7%) below the threshold for clinically significant change (6.42%) associated with osteoarthritis," the authors write. Furthermore, the network generalizability was extensively assessed using 389 additional knees from studies, with unique data distributions due to varied acquisition settings.
"This work shows the capability of deep learning to extract additional diagnostic information from already acquired T2 maps. With further development, a pipeline like this creates new possibilities for population studies like the OA Initiative, which can add to the characterization of OA, potentially facilitate clinical translation, and complement efforts to establish quantitative imaging biomarkers. Additionally, this study shows the promise of deep learning in accelerating imaging protocols through domain adaptation as opposed to more common reconstruction, standardization, and calibration approaches," writes Dr. Tong and co-authors.
Additional co-authors of the article include Aniket Tolpadi of the University of California San Francisco and the University of California Berkeley; Rupsa Bhattacharjee, Misung Han, Sharmila Majumdar, and Valentina Pedoia of the University of California San Francisco.
Read more about research and news at UCSF ci2.
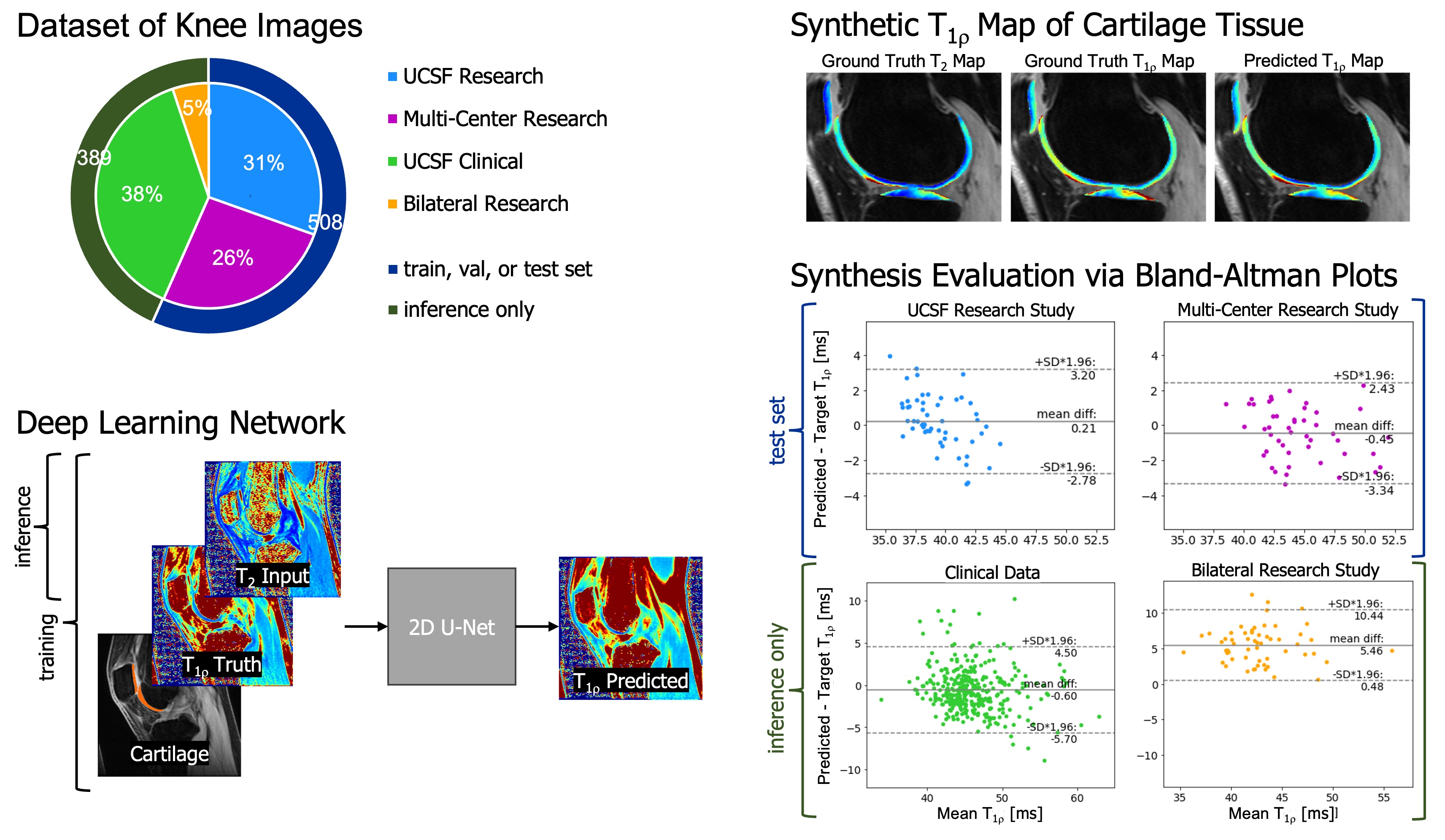
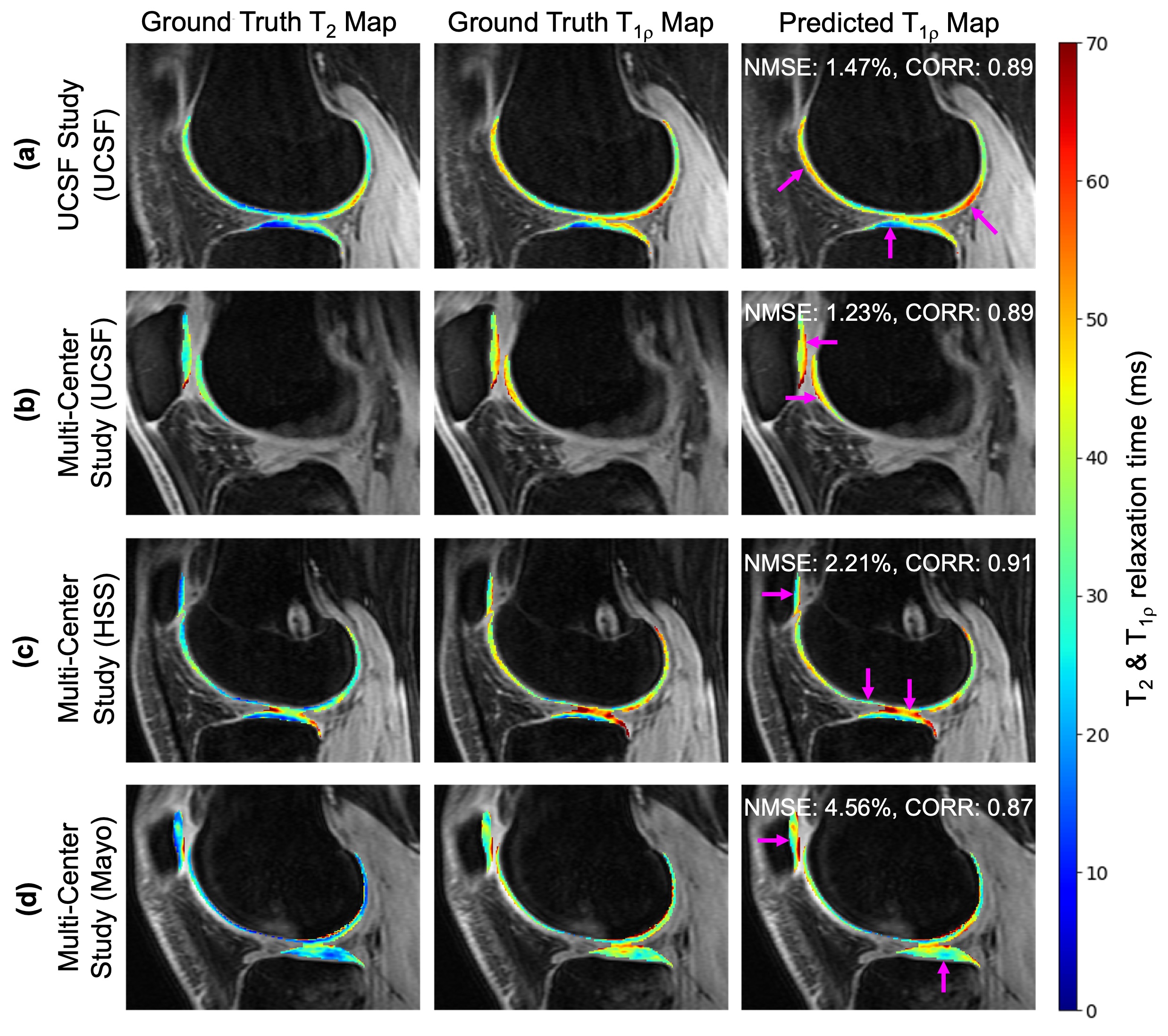