Clinical decision making to address spinal pain and functional disability relies on an accurate characterization of spinal deformities — but the current measurement methods are time-consuming, and the result is simplified to 2D, among other issues.
To address the limitations of current methods, a team including researchers from UC San Francisco (UCSF) Department of Radiology and Biomedical Imaging and the UCSF Center for Intelligent Imaging (ci2) developed 3D spinal curvature and global alignment parameters.
Investigators developed and validated a deep learning algorithm to "automatically extract the vertebral midline for frontal and lateral radiographs." The team share the findings in "Institution-wide shape analysis of 3D spinal curvature and global alignment parameters," which was published in the Journal of Orthopaedic Research.
"The resulting 3D dataset is used to describe global imbalance parameters in the patient population and to build a statistical shape model to describe global spine shape variations in preoperative deformity patients via eight interpretable shape parameters," write the investigators, led by Claudia Iriondo, PhD, a former graduate student researcher at UCSF.
The team used 20,118 pairs of full spine radiographs acquired over 12 years from 15,378 patients to develop the 3D spine approximations. Those results demonstrate "robust performance across datasets and patient populations" and will better represent the complexity of the spine – an articulated, 3D structure with 6 degrees of translational and rotational freedom.
"The developed method can identify patient subgroups with similar shape characteristics without relying on an existing shape classification system," conclude the investigators.
Additional authors from UCSF ci2 include senior author Sharmila Majumdar, PhD, Rutwik Shah, MD, Upasana Bharadwaj, MD, Emma Bahroos, Cynthia Chin, MD, and Valentina Pedoia, PhD.
Read more about research and news at UCSF ci2.
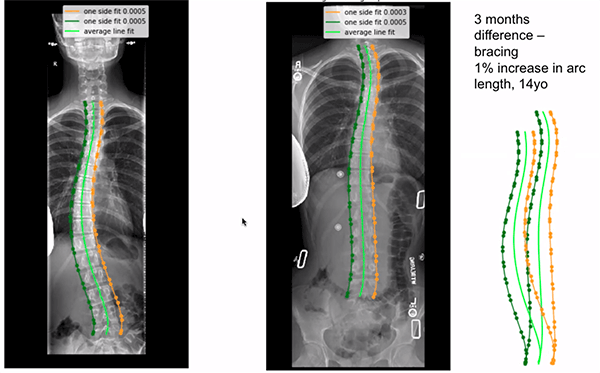
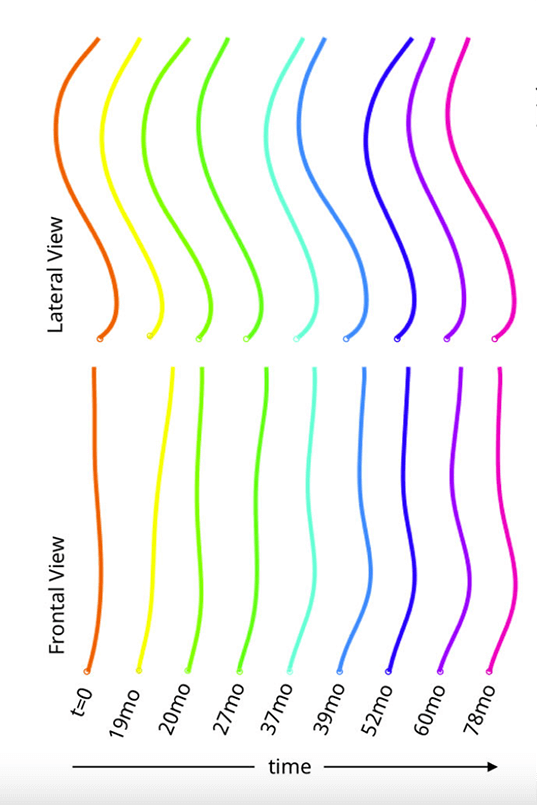