Contrast-enhanced MRI is an essential tool for helping to diagnose and monitor a variety of brain diseases. Unfortunately, the need for intravenous injection of gadolinium-based contrast agents (GBCAs) sometimes presents a barrier for patients who need brain MRI examinations for several reasons:
- While these contrast agents have a very strong safety profile, some patients may experience adverse or allergic reactions ranging from mild to life threatening.
- Some patient populations (such as pregnant women and those with severe chronic kidney disease) cannot safely receive these agents.
- There's also a growing body of evidence showing that gadolinium accumulates in the brain and bones of patients who have received multiple doses of MRI contrast and can persist for many years.
- The long-term consequences of gadolinium deposition are unknown, though no negative effects have been identified to date.
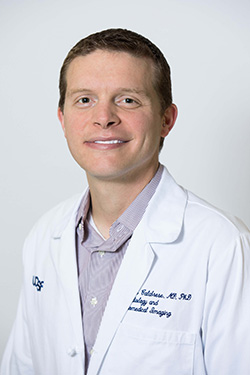
With this in mind, a team of investigators from UCSF Radiology and the UCSF Center for Intelligent Imaging (ci2) sought to simulate diagnostic quality contrast-enhanced MRI using only non-contrast MRI sequences. They evaluated the feasibility and accuracy of simulated post-contrast T1-weighted brain MRI generated from pre-contrast MR images in patients with brain gliomas. The research was just accepted by Radiology: Artificial Intelligence.
"We retrospectively analyzed MRI scans from 400 patients with brain gliomas including tumors with and without contrast enhancement," says Evan Calabrese, MD, PhD, radiology resident and corresponding author on this study. This was part of his T32 research fellowship. "We used a deep convolutional neural network to simulate post-contrast images using eight routine MRI sequences that were acquired before contrast was administered. We then evaluated the similarity of simulated versus real post-contrast images using quantitative image analysis and qualitative radiologist evaluation."
They found that simulated post-contrast images were highly similar to real post-contrast images both across the whole brain and within the tumor region using a variety of quantitative image similarity metrics. There was no difference in the ability of neuroradiologist readers to determine tumor grade in real versus simulated images (accuracy, 87.7% vs 90.6%; P = .87) and readers were not able to reliably distinguish real images from simulated images (accuracy, 59.1%, Cohen's Kappa, -0.23).
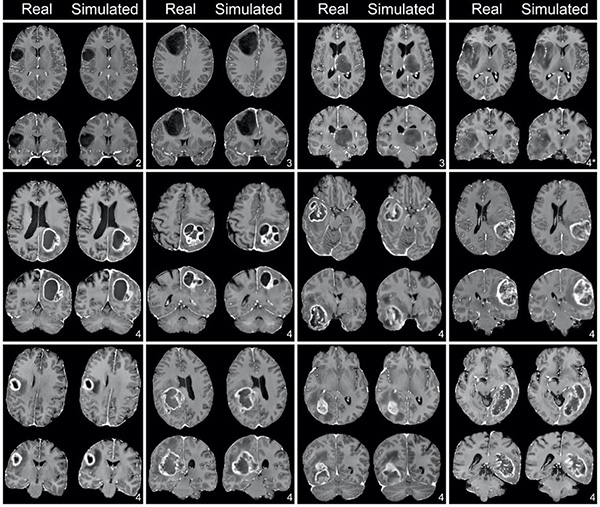
"We were able to generate quantitatively accurate and clinically convincing simulated post-contrast images using only routine non-contrast MRI series in patients with enhancing and non-enhancing brain tumors," says Dr. Calabrese. "With further study and validation, this technique could offer the benefit of a complete contrast-enhanced brain MRI examination to patients who cannot or prefer not to receive GBCA-based contrast agents."
Additional authors include Soonmee Cha, MD, vice chair of education at UCSF Radiology, Javier Villanueva-Meyer, MD and Andreas Rauschecker, MD, PhD faculty at UCSF Radiology and UCSF ci2 members and Jeffrey Rudie, MD, PhD, UCSF Radiology clinical instructor.
"We anticipate that this technology could help reduce the amount of GBCAs administered to patients who require multiple short interval follow up MRI examinations," says Dr. Calabrese.
This study employed a 3D fully convolutional deep neural network architecture with 24 convolutional layers and 4-dimensional MRI input (3D images plus multiple different MRI series). A major part of this project was creating, curating and processing an imaging database of almost 500 patients with brain gliomas who received preoperative MRI and subsequent tumor resection at UCSF. This database also includes detailed tumor genetic sequencing results (using a 500-gene panel for solid tumors developed at UCSF - the "UCSF 500"), which is being used in a separate project focused on using preoperative imaging to predict the presence of certain mutations in brain gliomas that affect prognosis or alter clinical management. This unique dataset is being shared publicly via the 2021 Radiological Society of North America (RSNA) annual Artificial Intelligence (AI) Challenge at this fall's Annual Meeting, for which UCSF will be the second largest data contributor.