New recommendations on diagnostic classification of diffuse gliomas requires an assessment of molecular features, that include IDH-mutation and 1p19q-codeletion status. The issue lies in that genetic testing requires an invasive, costly process that can often take weeks to get the results. An alternative noninvasive approach is also especially attractive if resection is not recommended. A team of UCSF investigators set out to improve the noninvasive classification of glioma genetic subtypes with deep learning and diffusion-weighted imaging. Their study was recently published in Neuro-Oncology, the journal for the society of Neuro-oncology (SNO).
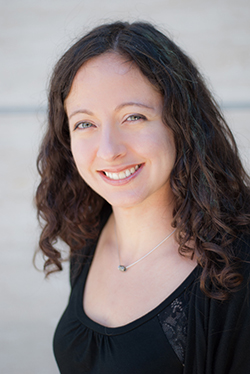
"The goal of our study was to evaluate the effects of training strategy and incorporation of biologically relevant images on predicting genetic subtypes with deep learning," says Janine Lupo, PhD, corresponding author on this study. As a member of the UCSF Center for Intelligent Imaging (ci2), Dr. Lupo's research focuses on the development and application of novel MRI data acquisition, processing, and analysis techniques for the evaluation of patients with brain tumors and other neurological diseases using our research 3T and 7T whole body scanners.
The research dataset consisted of 384 patients with newly diagnosed gliomas who underwent preoperative MRI with standard anatomical and diffusion-weighted imaging, and 147 patients from an external cohort with anatomical imaging. The team used tissue samples acquired during surgery to classify each glioma into one of the three subgroups:
- IDH-wildtype (IDHwt)
- IDH-mutant/1p19q-noncodeleted (IDHmut-intact)
- IDH-mutant/1p19q-codeleted (IDHmut-codel)
From there, the team optimized training parameters, including whether or not to pre-train on Google's ImageNet data, and determining the best approach for incorporating diffusion-weighted imaging data. Then, top performing convolutional neural network (CNN) classifiers were trained, validated, and tested using combinations of anatomical and diffusion MRI with either a three-class or tiered structure that first predicted the IDH mutation, followed by 1p19q codeletion status for IDH-mutant tumors. Generalization to an external cohort was assessed using anatomical imaging models. The best model used a 3-class CNN containing diffusion-weighted imaging as an input, achieving 86% (95% CI: [77, 100]) overall test accuracy and correctly classifying 95%, 89%, 60% of the IDHwt, IDHmut-intact, and IDHmut-codel tumors.
"Our results show that diffusion imaging included as a modality can help improve the accuracy of deep learning classification into IDHwt, IDHmut-codel, and IDHmut-noncodel genetic subtypes of glioma," says Julia Cluceru, first author on this study who recently graduated from Dr. Lupo's lab earlier this year and is currently working as a Clinical Imaging Scientist at Genentech.
UCSF ci2 members that were a part of this study include Valentina Pedoia, PhD, Beck Olson and Javier Villanueva-Meyer, MD. Authors from UCSF Radiology and Biomedical Imaging include Tracy Luks, PhD research specialist and clinical research coordinators Marisa Lafontaine and Devika Nair. Authors from UCSF Neurological Surgery include Susan Chang, MD, Joanna Phillips, MD, PhD and Annette Molinaro, MD, PhD (faculty) and Anny Shai (clinical research analyst). Additional authors include, Pranathi Chunduru, former data science analyst at UCSF, now with Johnson & Johnson; Paula Alcaide Leon, former UCSF research specialist, now an assistant professor at the University of Toronto and Yannet Interian, assistant professor in the Master's in Data Science program at the University of San Francisco.
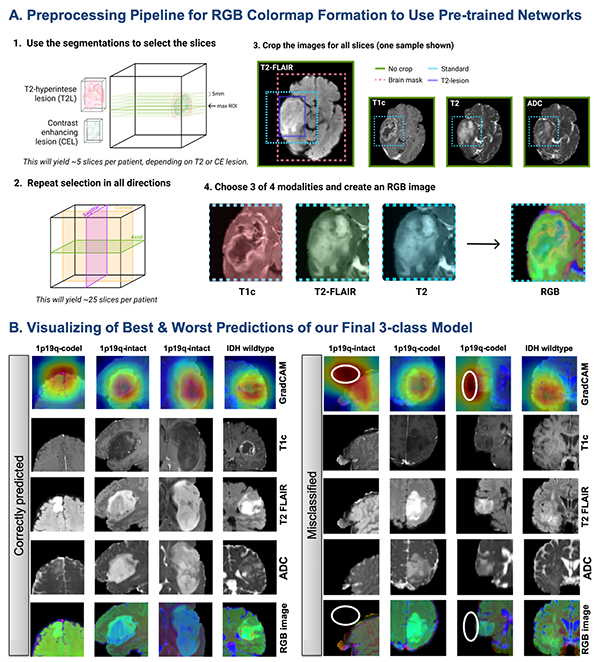