Principal author
Contributors
Sharmila Majumdar, PhD, is leading a new technology development grant from the NIH, as part of the HEAL Initiative and BACPAC consortium, aimed at improving treatments for chronic pain and curbing the rates of opioid use. Dr. Majumdar and a highly-experienced multidisciplinary research team – including UCSF’s Valentina Pedoia, PhD, Cynthia Chin, MD, Duygu Tosun, PhD, Irina Strigo, PhD, and Harvard’s Mary Bouxsein, PhD – bring together extensive expertise in MR bioengineering, advanced MRI data analysis, radiology, neuroscience, neurosurgery, orthopedic surgery, and multi-dimensional analytics to respond to the criticial need for clarity in the etiologies of chronic back pain and other disorders of the spine. Long missing reliable methods for determing the appropriate course of patient care and objectively evaluations of the effectiveness of various interventions, their project is leveraging key technical advancements to address this important problem. They are developing machine learning-based, faster MR acquisition methods as well as machine learning for image segmentation and extraction of objective disease-related features from images. Furthermore, the team will develop, validate, and deploy end-to-end deep learning-based technologies for accelerated image reconstruction, tissue segmentation, and detection of spinal degeneration to facilitate automated, robust assessment of structure-function relationships between spine characteristics, neurocognitive pain response, and patient-reported outcomes.
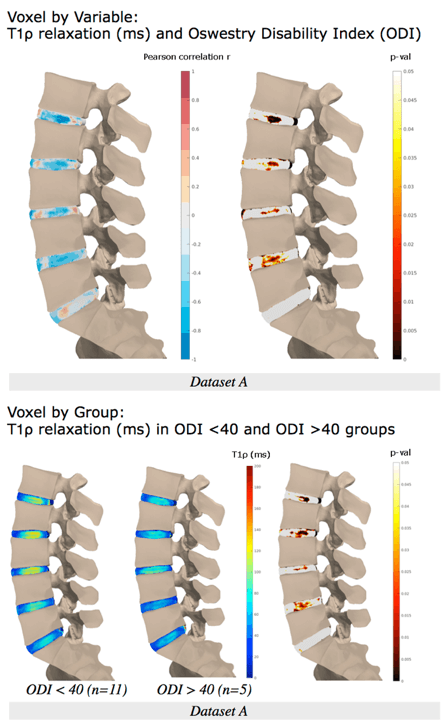
Bottom: Voxel by ground analysis, mean relaxation T1ρ maps and p-values for significant differences between minimal/moderate (0-40) and severe disability (>40) groups.