In a hospital or health system setting, the time taken from a clinician ordering imaging to radiology scan interpretation time is an important quality measure that impacts hospital workflow and patient outcomes. Delays in performing or interpreting radiology scans prolongs the time until a proper treatment decision can be made. This could lead to increased costs and potential compromises in patient care.
Here at UC San Francisco, the Big Data in Radiology (BDRAD) research team – part of the UCSF Center for Intelligent Imaging (ci2) – noted that during non-businesses hours, the scenario presented could be especially impactful. Predicting whether a delay can occur after a radiological exam request has a number of benefits for clinicians, radiologists, residents and educators. They developed a machine learning model – aided by natural language processing (NLP) components – to predict delayed turnaround time during non-business hours and identify factors that contribute to this delay.
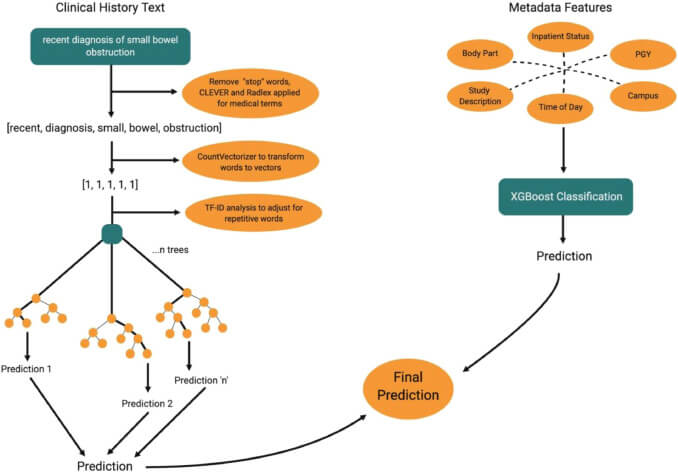
To perform the research, they used over 15,000 CT exams from both UCSF Medical Center and the Zuckerberg San Francisco General Hospital and Trauma Center (ZSFG) to train and evaluate the algorithm. When testing, the model achieved an area under the curve (AUC) of 0.85 for predicting longer-than-average turnaround times for on-call radiology exams. Findings were recently published in Academic Radiology.
“Using NLP components that could predict delay in interpretation of on-call radiology cases, followed by identification of the factors that contributed most to the prediction allows other institutions to adopt and train similar models using our established approach,” says Jae Sohn, MD, MS, senior author, UCSF ci2 and UCSF Radiology faculty member and co-founder of the BDRAD research team.
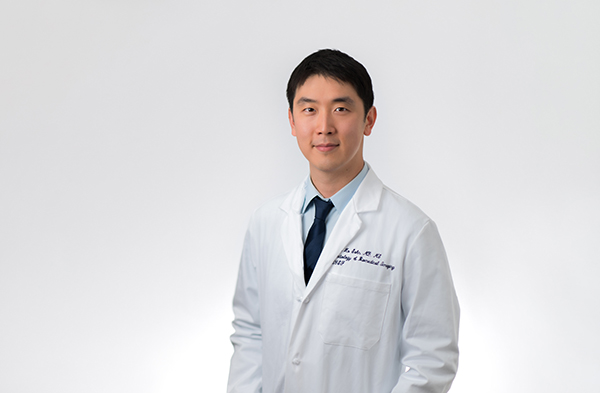
“Important factors used by the model included body part, hospital campus where the scan was ordered, inpatient status of the patient, PGY level in training of the radiologist, study description of the scan, time of day when the scan was conducted and written clinical history,” says Dr. Sohn. “An important value of the model is that it can measure past quality in the radiology workflow giving health systems an opportunity to reveal areas that may benefit from a formal quality improvement process.”
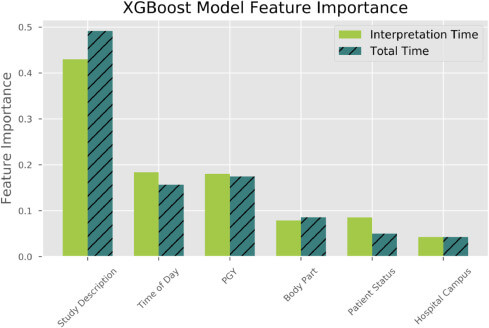
First author on this study was Vaibhavi Shah, aspiring surgeon and scientist who recently began her first year of medical school at Stanford University. Yeshwant Chillakuru, MSc is a BDRAD team member that played a key role in development of the algorithms. Youngho Seo, PhD, Thienkhai Vu, MD, PhD, and Alex Rybkin, MD at UCSF ci2 and/or UCSF Radiology faculty members, played key mentoring and supervisory roles.
“This algorithm can be applied clinically when a physician is ordering the scan to reasonably predict delayed turnaround time,” write the team of investigators. “Such a model can be leveraged to identify factors associated with delays and emphasize areas for improvement to patient outcomes.”
Overall, the team sees this work as a starting point for future QI projects. Additional variables that could be added include average technology operating time, hospital and emergency department (ED) patient census, number of providers and transportation.