In typical or standard lung cancer screening, patients who meet the criteria for lung cancer screening (mainly based on age and smoking history) will come in for a low-dose CT scan, and the scan is reviewed by a radiologist to see if there are any indications of cancer or other clinically significant findings. There is a significant component of manual analysis, but now in the era of artificial intelligence (AI), more automatic algorithms are being used to analyze these CT scans.
"At this point, the analysis of the scan is not completely automated. It is still done with humans being mainly in the driver's seat. But we use certain types of nodule detectors, and nodule analyzers, sometimes in conjunction with companies, and sometimes in conjunction with some of the research algorithms that are developed in the community, including at UCSF," says Jae Ho Sohn, MD, MS, UCSF Center for Intelligent Imaging (ci2) member and UCSF Radiology faculty member in the Cardiac and Pulmonary Imaging Section. One of Dr. Sohn's areas of research is AI and lung cancer screening.
According to Dr. Sohn, there are at least three benefits to using AI in lung cancer screening:
1. Increased nodule detection rate. "The AI algorithm will miss nodules that humans will detect, but it also detects nodules that humans may miss. So together as a team, in a process called ensemble approach, the overall nodule detection rate would increase.," Dr. Sohn explains.
2. More objective and precise measurement of nodules. "When an AI algorithm detects nodules and puts a caliper on the size of the nodules, it's less prone to precision errors from the ruler being offset a little bit. Also, algorithms will tend to be much more consistent each time compared to a group of human radiologists. This results in more accurate nodule size reporting." says Dr. Sohn
3. The ability to automatically extract information that humans don't necessarily clinically extract each time we interpret CTs. "This information may include things such as what is the patient's general health? What is the patient's level of coronary artery disease? What is the patient's level of emphysema and lung health?" says Dr. Sohn. These are some examples and there are more. Not all of these functions are used clinically within our system right at the moment, but these are things that are pretty far along in development, some of which are already starting to be made available to extract as much useful information as possible from lung cancer screening.
AI, just like any new technology when introduced, can have inherent inefficiency with the workflow. But according to Dr. Sohn, as the technology develops and there's more trust, there may be opportunities to speed up the work.
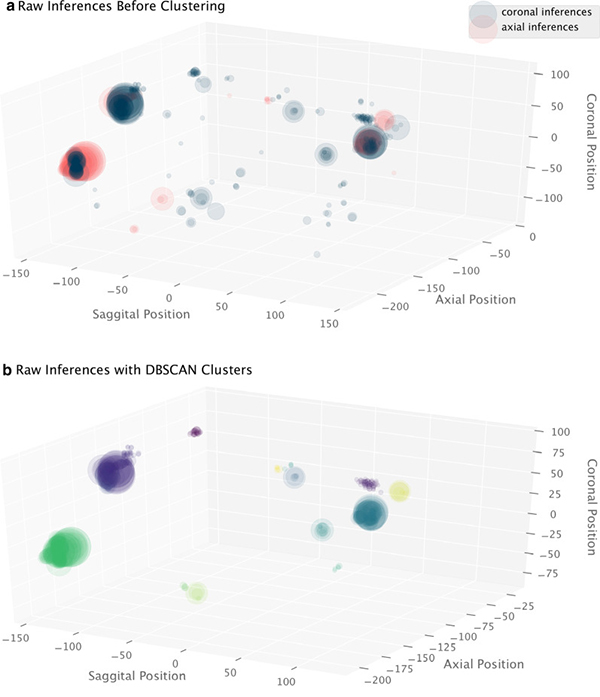
Dr. Sohn's recent published research involves high precision nodule detection. "I've worked on a high precision nodule localizer, using an algorithm based on the RetinaNet," says Dr. Sohn. "It's a type of deep learning algorithm, and it is specifically optimized for detection of tiny objects in a very large space, like the lungs. Typical AI algorithms are not optimal for detecting these tiny objects. They're better for classifying a big object in an image. What's unique about this algorithm is its high precision, meaning that almost all nodules detected by the algorithm were real nodules and not false positives."
After the breakthrough, his team was able to use this algorithm, run it on local databases, as well as some of the publicly available datasets from clinical trials and have started creating a very large database of all the nodules that are detectable on chest CTs. They are also now working on these nodules to answer the question - what makes nodules, more suspicious for cancer or less suspicious for cancer? According to Dr. Sohn, there are known criteria well published in the literature that established the importance of nodule size and shape, but two other important variables are not as easily captured in automated nodule analysis algorithms:
- Is the nodule growing and at what pace? "We're now integrating a temporal/longitudinal component into the artificial intelligence to compare two scans of nodules and see -- is this changing and changing at a pace consistent with cancer?" he says.
- What is going on or around the nodule is actually just as important as the nodule itself. If there are signs of infection around nodules in the lungs, that actually greatly reduces the probability of the nodule becoming cancerous. "We're using AI to try to capture that peripheral neighborhood element as well as patient's profile to categorize it into more precise and personalized risk stratification. That's the other sort of research direction that we're taking," says Dr. Sohn.
What's next for the future of AI and lung cancer screening? Check back with us soon to hear more from Dr. Sohn.