Low back pain is a leading cause of disability worldwide, and while nociceptive source in most cases cannot be identified, there has been a growing collection of evidence showing that properties of vertebral endplates are closely linked to intervertebral disc degeneration and lower back pain.
Modic changes (MCs) are known as the most prevalent classification system for describing MRI signal intensity changes in the vertebrae. Right now, there is a growing need for novel quantitative and standardized methods of characterizing these anomalies, in particular for lesions of transitional or mixed nature, based on a lack of conclusive evidence of their associations with low back pain.
With all of this in mind, investigators at the UCSF Center for Intelligent Imaging (ci2), conducted a retrospective imaging study with the goal of developing an interpretable deep learning-based detection tool for voxel wise mapping of MCs. The study was recently published in JOR Spine, a fully open access title from the Orthopaedic Research Society (ORS).
"We enrolled 75 lumbar spine MRI exams that presented with acute to chronic low back pain, radiculopathy, and other symptoms of the lumbar spine, and our pipeline consists of two deep convolutional neural networks to generate an interpretable voxel wise Modic map," says Kenneth Gao, PhD candidate at UCSF who developed and trained the deep learning models along with the UCSF ci2's Radhika Tibrewala and Madeline Hess.
The next steps in the methodology were as follows:
- First, an encoder-decoder network was trained to segment vertebral bodies from T1 weighted sagittal lumbar spine images.
- Next, two radiologists segmented and labeled MCs from a combined T1 and T2 weighted assessment to serve as ground truth for training a second encoder-decoder that performs segmentation of MCs.
- The voxels in the detected regions were then categorized to the appropriate Modic type using a rule-based signal intensity algorithm.
- Post hoc, three radiologists independently graded a second dataset with the aid of the model predictions in an artificial (AI) assisted experiment.
"Overall, our novel, deep learning-based approach demonstrates a substantial agreement with radiologists and may serve as a tool to improve inter rater reliability in the assessment of Modic changes," says Gao.
Additional authors from the UCSF ci2 include Upasana Bharadwaj, MBBS, Cynthia Chin, MD, Valentina Pedoia, PhD and Sharmila Majumdar, PhD who contributed to the study design along with Thomas Link, MD, PhD and Gaurav Inamdar.
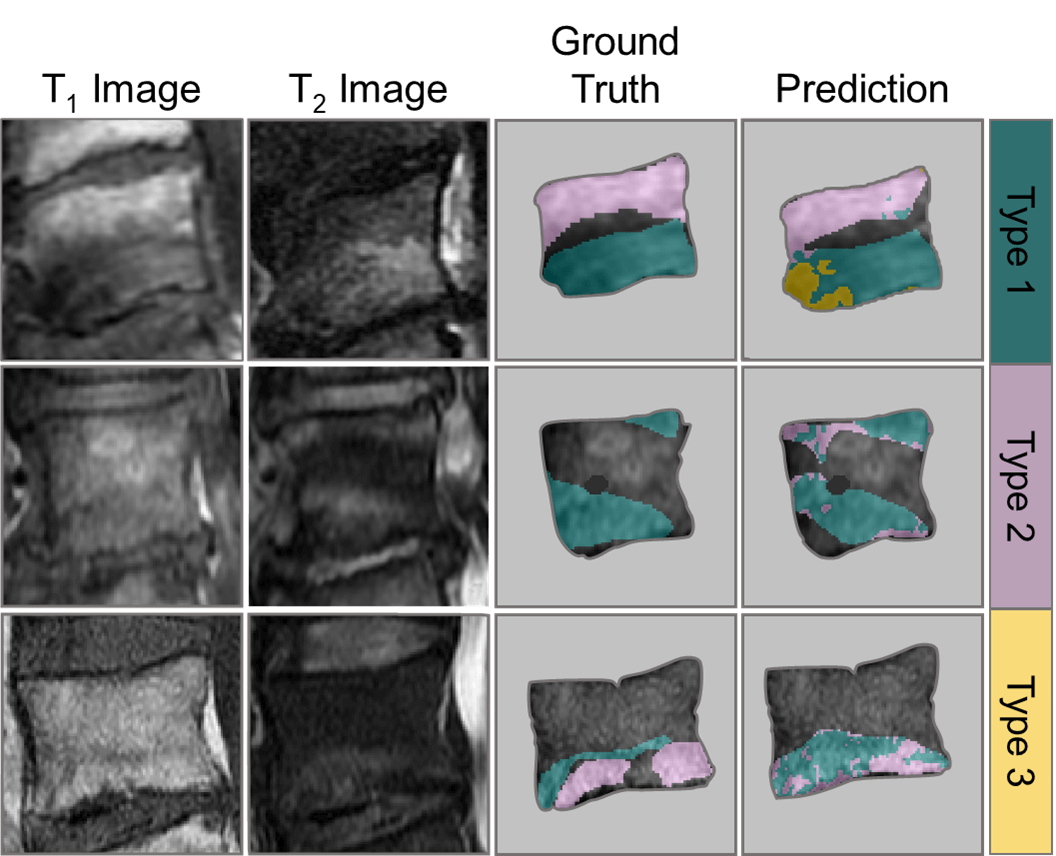