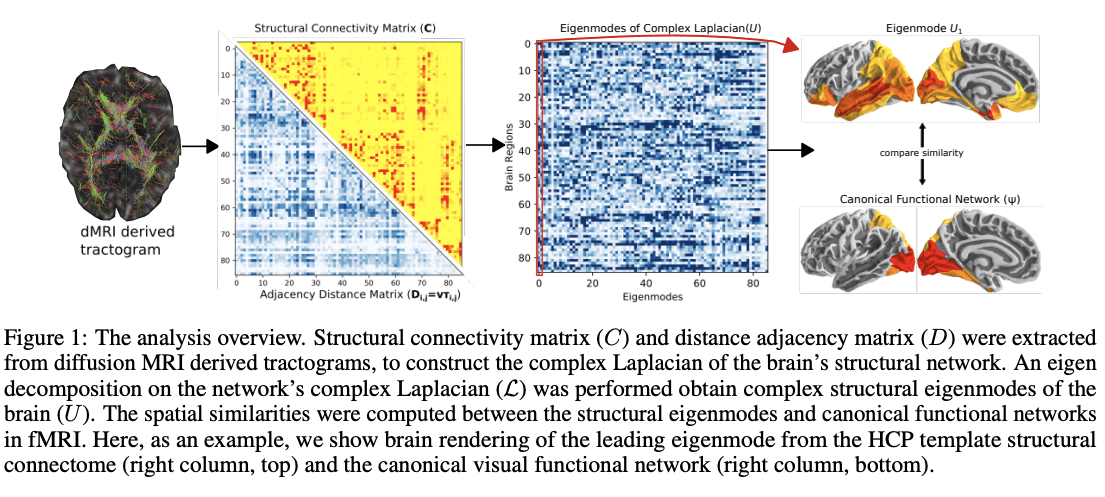
How do functional brain networks emerge from the underlying wiring of the brain? This was a question asked by a team of investigators from the UCSF Center for Intelligent Imaging (ci2) and UCSF Radiology. They examined how resting-state functional activation patterns emerge from the underlying connectivity and length of white matter fibers that constitute its "structural connectome," a key determinant of brain function and dysfunction. Their paper was recently published in NeuroImage (open access here).
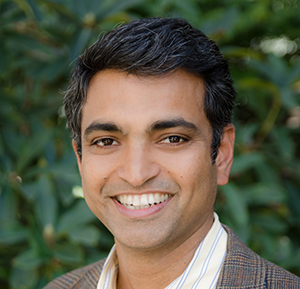
In computational neuroscience, the relationship between the brain's structural wiring and the functional pattern of neural activity is of fundamental interest. As director of the Biomedical Imaging Laboratory (BIL) at UCSF Radiology and ci2 member, Srikantan Nagarajan, PhD and his team – among other goals – are interested in developing machine learning algorithms and tools for functional brain imaging and imaging brain connectivity. Dr. Nagarajan's team collaborates with Ashish Raj, PhD, ci2 member and director of the Brain Networks Laboratory at UCSF Radiology. Dr. Raj's lab focuses on understanding the mechanisms of healthy and diseased brains by applying computational tools to neuroimaging data.
Data from neuroimaging such as diffusion-weighted and functional magnetic resonance imaging (dMRI and fMRI) can help reveal important relationships between the brain's structure and function and using creative models can help to really bridge the gap between empirical data and theories about the brain. In this work, the team shares that complex valued network Laplacians - obtained by introducing realistic signal transmission delays along fiber projections - explain how functional brain networks emerge from underlying wiring of the brain. Using a novel parameter inference procedure, they show that the complex Laplacian outperforms the real-valued Laplacian in predicting functional networks.
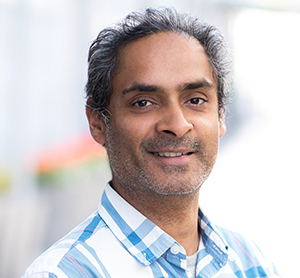
"The introduction of the complex-valued Laplacian and accompanying complex graph diffusion may be an important contribution to the emerging literature on graph models of brain activity and furthers our understanding of the structure-function relationship in the human brain," say the authors.
Pablo Damasceno, PhD, a computational and data scientist in the ci2, played a key role in this work. We recently wrote about the Computational Core, one of the five pillars within the ci2. They support researchers across UCSF including building computational infrastructure, data science tools, and platforms and providing core competency in machine learning and software development. As part of this specialized team, Dr. Damasceno helped develop software for easier parcellation of the brain into commonly known areas.
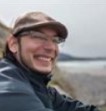
"This allowed the team to digest their data more easily, and also better compare their results with other papers," says Dr. Damasceno. "Without this method, we would be looking at tens of thousands of variables related to every millimeter of the brain, but with this method which averages the data per brain 'parcel' – we can reliably explain what is happening in each of these [roughly 100] regions. In other words, the algorithm translates coordinates into brain regions that we can pronounce."
Xihe Xie, visiting graduate at UCSF from Weill Cornell Neurosciences (and member of the Brain Networks Lab) wrote the original draft of this work and was a contributor along with Chang Cai, postdoctoral researcher, and member of the BIL at UCSF Radiology.