It is known that artificial intelligence (AI) has great potential across many specialties in radiology. However, up until recently, it had not been used to create differential diagnoses for rare and common diseases at brain MRI. Since a majority of MRIs conducted each year are to assess the central nervous system (CNS), the demand for such work has become increasingly important.
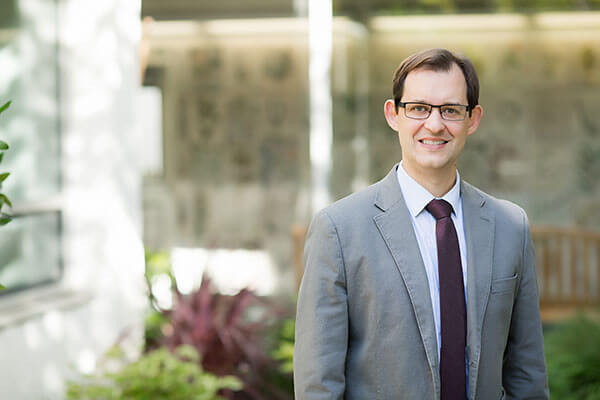
As a neuroradiologist and assistant professor, Andreas Rauschecker, MD, PhD’s research focuses on using AI and advanced image-processing techniques to investigate the brain and its appearance in relation to disease. He, along with Jeffrey Rudie, MD, PhD (clinical instructor at UCSF Radiology), Michael Tran Duong, MD (Perelman School of Medicine, University of Pennsylvania) and others develop an automated, deep learning-based method for segmentation of abnormalities on FLAIR (fluid-attenuated inversion recovery) - a sequence that is part of almost all protocols for imaging the brain. The performance of the algorithm was high across a variety of underlying pathologies and image acquisition parameters. Their work was published in the American Journal of Neuroradiology (AJNR).[1]
A fully automated, rapid, precise, quantitative assessments of FLAIR abnormalities can standardize quantitative descriptions of pathology and has many benefits including reducing errors from interobserver variability, improvement of workflow, efficiency, and diagnostic accuracy, ultimately perhaps leading to better patient outcomes.
From there, Dr. Rauschecker and team conducted a set of retrospective studies to test performance of two AI systems for probabilistic diagnosis in patients with a total of 50 separate common and rare diagnoses at brain MRI. The AI system combined deep learning, including the FLAIR abnormality detection algorithm described above, feature engineering, and an expert-knowledge-derived Bayesian network to fully computationally model the steps used by a neuroradiologist reading brain MRIs. They found that these AI systems provided differential diagnoses at the level of academic neuroradiologists and exceeded that of less-specialized radiologists. This research was published in two publications in Radiology and Radiology: Artificial Intelligence.[2]
Overall, such findings demonstrate a step forward for a system aimed at diagnosing brain diseases that combines AI methods with the expertise of human radiologists. Furthermore, it emphasizes the need for AI systems to not only provide diagnoses but to also characterize lesions on complex imaging such as brain MRIs.
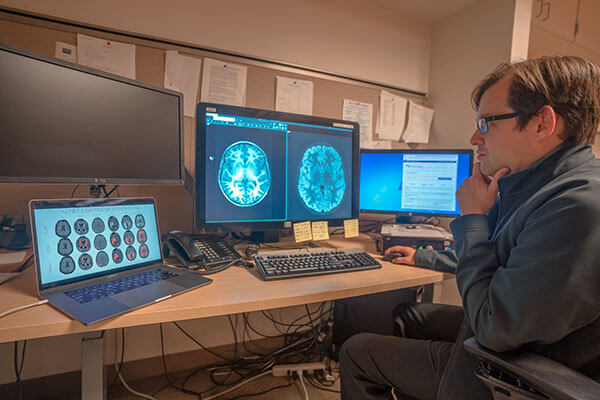
[1] Dr. Rauschecker was corresponding author on this study. Drs. Duong and Rudie contributed equally to this work and are co-first authors. The full list of authors includes Jiancong Wang, Long Xie, Suyash Mohan, MD, PDCC and James C Gee from the Department of Radiology, Hospital of the University of Pennsylvania.
[2] Dr. Rauschecker was corresponding author on this study. Dr. Rudie contributed equally to this work. The full list of authors includes Jiancong Wang, Long Xie, Suyash Mohan, MD, PDCC, James C Gee, Asha M. Kovalovich, MD, John Egan, DO, Tessa S. Cook, MD, PhD, CIIP and Ilya M Nasrallah, MD PhD from the Department of Radiology, Hospital of the University of Pennsylvania; Emmanuel Botzolakis, MD from Mecklenburg Radiology Associates and R. Nick Bryan, MD from the Department of Radiology, University of Texas at Austin.