Each year the International Conference on Medical Image Computing and Computer Assisted Intervention (MICCAI) host a variety of workshops, challenges, and tutorials. Challenges have become an integral part of MICCAI conferences in the past years. This year, the UCSF Center for Intelligent Imaging (ci2) hosted a challenge titled K2S: from under sampled K-space to automatic Segmentation.
There were two major problems in this competition - a problem of under sampled volumetric k-space and a problem of 3d segmentation of volumetric medical images. The goal of the K2S-challenge was to find an accurate segmentation for under sampled knee MRI data. Teams were tasked with developing segmentation models for anatomical structures (cartilage, bone) from 8x under sampled, multi-channel k-space data of 3D FSE-Cube MRI sequences. Let’s congratulate and highlight the winners of this year’s challenge and provide a short overview of their work.
First place - RegSeg - combining image regression with segmentation
Jan Nikolas Morshuis, Paul Fischer, Matthias Hein and Christian Baumgartner from the University of Tubingen introduced RegSeg, a method that combines the regression problem of blurry image-reconstruction with segmentation. The RegSeg architecture consists of two parts. The first part is a regression network that has been trained to reconstruct the fully sampled image given the under sampled image. The second part consists of a segmentation network that predicts a segmentation from the reconstruction as well as the under sampled image.
Second place - Volumentic segmentation from undersampled k-space
Artem Razumov and Dmitry V. Dylov from the Skolkovo Institute of Science and Technology created a solution that has two main parts: a method of reconstruction from under sampled k-space and deep learning model for 3d image segmentation.
Third place - a fully automated end-to-end pipeline for generating high-resolution multi-class knee segmentations from undersampled k-space
Stephen Fransen and Quintin van Lohuizen from the University Medical Center Groningen submitted a fully automated end-to-end pipeline for generating high-resolution multi-class knee segmentations from under sampled k-space. Their system can simultaneously segment large bones and small cartilages with high accuracy. Their proposed method leveraged patch-based self-ensembling to increase the stability of the predictions and uses a sliding window to generate high-resolution predictions of varying sizes. Their pipeline consisted of three main steps: K-space reconstruction, deep learning-based segmentation, and misclassification removal.
Fourth place - using deep learning-based approach for knee segmentation from under-sampled data
Xiaoxia Zhang, Marcelo Zibetti, Hector De Moura, Radhika Tibrewala, Kaning Liu and Ravider Regatte from the NYU School of Medicine created a two-step method consisting of deep learning-based (DL-based) reconstruction and the DL-based segmentation. The fully sampled k-space data is under-sampled by applying the provided mask templates. The under-sampled data is separated into slices for reconstruction. Using ESPIRiT, they obtained coil sensitivity maps and under-sampled images. The fully sampled k-space data is also reconstructed to images using ESPIRiT and are used as targets to train the network.
Overall, submissions were evaluated in an end-to-end fashion from under sampled k-space data to segmentation on a test set of 50 fat-suppressed FSE-Cube sequences (under sampling pattern same as training data). Congratulations to the winners and thank you to all teams who participated. We will see you all again next year.
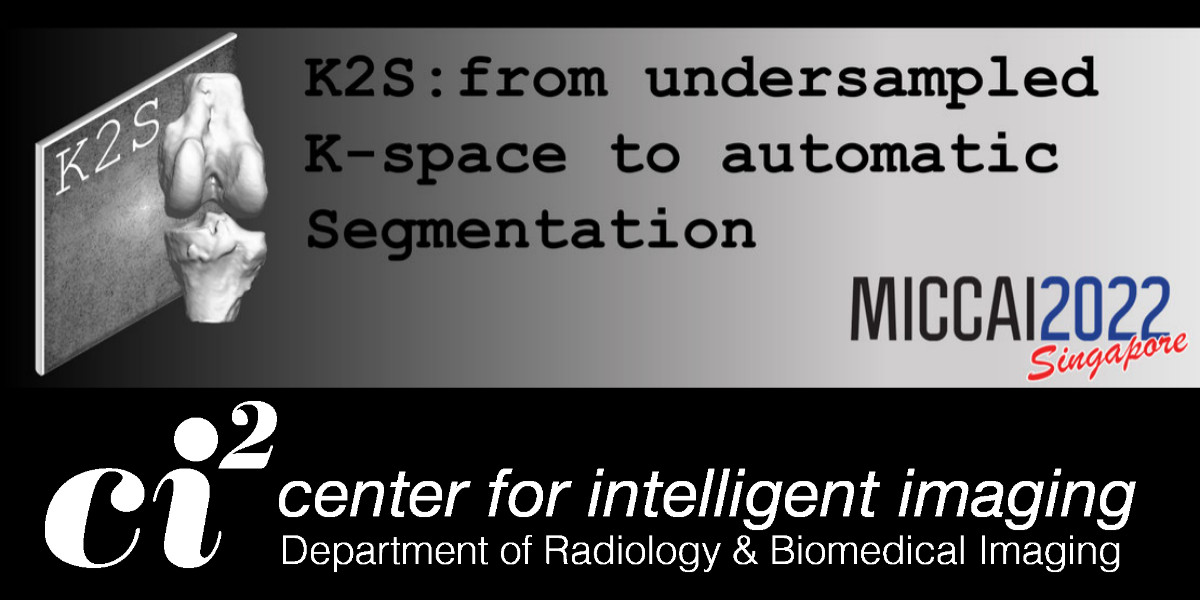