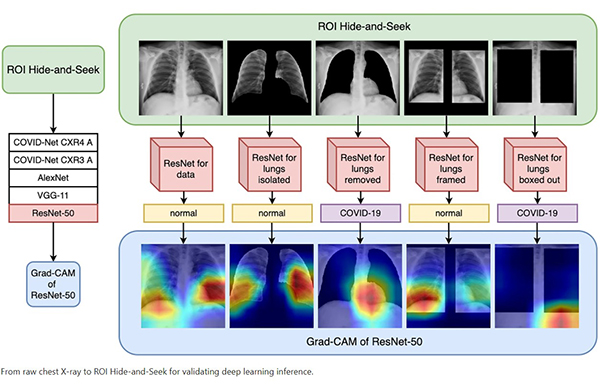
As the number of COVID-19 infections soared in spring 2020, chest X-ray (CXR) imaging became more relevant in the early diagnosis and treatment planning for patients with suspected or confirmed COVID-19 infection. In a few weeks, proposed new methods for lung screening using deep learning rapidly appeared.
A recently published paper from the Lawrence Berkeley National Laboratory, UCSF Center for Intelligent Imaging (ci2), the Bakar Computational Health Sciences Institute (BCHSI), UC Berkeley Institute for Data Science and the Thomas Jefferson University Hospital Department of Radiology proposes a set of protocols to validate deep learning algorithms, highlights potential gaps in using neural networks for analyzing these x-rays, including a region of interest Hide-and-Seek protocol, which emphasizes or hides key regions of interest from CXR data.
"Our protocol allows assessing the classification performance for anomaly detection and its correlation to radiological signatures, an important issue overlooked in several deep learning approaches proposed so far," say the authors. "By running a set of systematic tests over CXR representations using public image datasets, we demonstrate the weaknesses of current techniques and offer perspectives on the advantages and limitations of automated radiography analysis when using heterogeneous data sources."
Full findings can be found in Scientific Reports.
"We sought to answer a number of answered questions related to CXR imaging for COVID-19 screening," says Sharmila Majumdar, PhD, UCSF ci2 executive and scientific director and a co-author on this paper. "Our paper investigates the role that the lung segmentation might play in the CXR classification process, particularly when including the dataset includes source data from previously known respiratory infection cases and COVID-19 specific imaging."
Senior author on this study was Daniela Ushizima, staff scientist, Computational Research Division at Berkeley Lab and affiliate faculty of UCSF BCHSI. Additional authors include lead Robbie Sadre, machine learning research engineer with the Berkeley Lab and Baskaran Sundaram, MD, professor of radiology and director of the Division of Cardiothoracic Radiology at Thomas Jefferson University Hospital.
The datasets generated during and/or analyzed during the current investigation are available in the github repository.