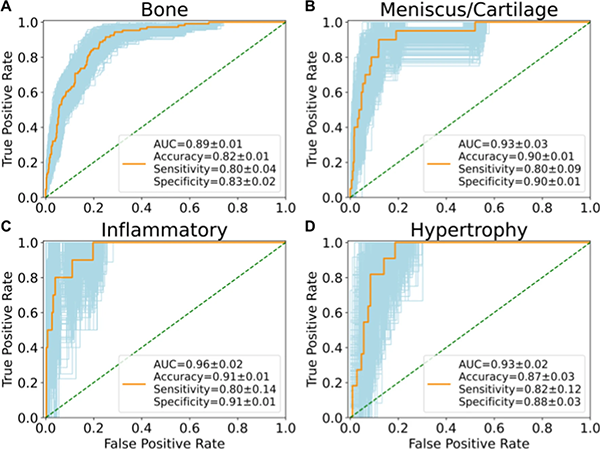
Osteoarthritis (OA) is the most common form of arthritis, affecting 32.5 million U.S. adults. It is caused by breakdown or damage of joint cartilage between bones, and currently there is no cure. At this time, doctors treat OA through a combination of therapies. Because OA develops through heterogenous pathophysiologic pathways, no regulatory agency approved disease modifying OA drugs (DMOADs) are available to date.
"Stratifying knees into MRI-based morphological phenotypes may provide insight into predicting future OA incidence, leading to improved inclusion criteria and efficacy of therapeutics," hypothesized a team of investigators from the UCSF Center for Intelligent Imaging (ci2) including Nikan Namiri, medical student at UCSF School of Medicine, Sharmila Majumdar, PhD, Valentina Pedoia, PhD, UCSF Radiology faculty, and Rutwik Shah, MD, Felix Liu, Bruno Astuto Arouche Nunes, PhD and Jinhee Lee.
They set out to (1) build a fully automatic end-to-end deep learning model to stratify knees into pre-defined Rapid OsteoArthritis MRI Eligibility Score (ROAMES) phenotypes and (2), evaluate the prevalence and association of phenotypes with knee OA to better inform patient selection in clinical trials. They trained convolutional neural networks (CNNs) to classify bone, meniscus/cartilage, inflammatory, and hypertrophy phenotypes in knee MRIs from participants in the Osteoarthritis Initiative (OAI) (n = 4791).
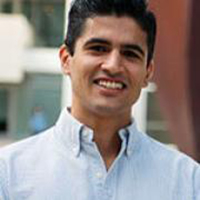
"Our end-to-end deep learning model rapidly stratified knees into MRI-based morphological phenotypes using a large, longitudinal cohort to examine odds of obtaining OA and total knee replacement (TKR) surgery," says Nikan Namiri. The full study – including materials, methods, results and discussion – was recently published in Scientific Reports.
Despite relatively satisfactory performance metrics from the CNNs, methods using deep learning are limited say the team in their discussion. Artificial intelligence (AI) can serve as a valuable aid for clinicians and researchers with high workload or limited expertise, but detailed evaluation of relevant pathology by radiologists is inevitably necessary for accurate staging and diagnosis.
Overall, their study emphasizes the value of morphological phenotypes for characterizing progression of knee OA. Findings hold implications for improving understanding of OA pathogenesis, which may guide inclusion criteria of DMOAD trials towards MRI-based structural phenotypes. This could improve effectiveness of DMOADs by using individual knee phenotypes to offer patient-specific treatment. Future research directions could include surveying individual DMOAD trials to analyze whether specific subgroups of structural phenotypes received increased therapeutic benefits.