What happens when a brain MRI lesion segmentation model was trained on a single institution's data, but then it performed lower when applied at a second institution? Investigators from UCSF Radiology and Penn Radiology sought to figure out why and also assess the effect of multi-institutional training datasets for mitigating performance loss. Findings were recently published in Radiology: Artificial Intelligence.
"Our paper looked at interinstitutional portability of a deep learning brain MRI lesion segmentation algorithm," says Andreas Rauschecker, MD, PhD, corresponding author and UCSF Center for Intelligent Imaging (ci2) and UCSF Radiology faculty member.
A three-dimensional U-Net for brain MRI abnormality segmentation was trained on data from 293 patients from one institution (University of Pennsylvania), and it accurately segmented lesions across various pathologic conditions. The performance was lower when tested at an external institution (UCSF) with a median Dice score of 0.70 for institution two [IN2] vs 0.76 for institution one [IN1]. From there, they found that an addition of 483 training cases of a single pathologic condition, including from IN2, did not raise performance. However, addition of training data from IN2 with heterogeneous pathologic features, representing only 10% (34 of 329) of total training data, increased performance to baseline (Dice score, 0.77; P < .001). Results showed that this final model produced total lesion volumes with a high correlation to the reference standard (Spearman r = 0.98).
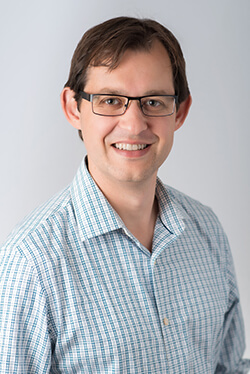
"The lesson we learned was that If you're smart about what (re-)training data you use, then you don't have to fret if your algorithm doesn't generalize as well as you'd like," says Dr. Rauschecker. "Ultimately, you want data where the training data closely match the image statistics of the target test data – the closer your fine-tuning data are in terms of MR acquisition parameters, lesion size/distribution/signal/numbers, and perhaps patient demographics, the more successfully you can apply your final model to the new setting, and the less data you need for re-training."
Overall, the investigators were able to conclude that adding a modest amount of relevant training data from an external institution to a previously trained model can support successful application of the model to this external institution.
Christopher Hess, MD, PhD, UCSF ci2 founding director was also an author. Authors from UCSF Radiology include Leo Sugrue, MD, PhD (associate professor), Evan Calabrese, MD, PhD and Tyler Gleason, MD (neuroradiology clinical fellows) and Pierre Nedelec, data science specialist. Former UCSF authors include John Colby, MD, PHD (current neuroradiologist at MarinHealth Medical Center) and Jeffrey Rudie, MD, PhD (current neuroradiologist at Scripps Health and UCSD Radiology faculty). Authors from Penn Radiology include Michael Tran Duong and Davis Weiss.