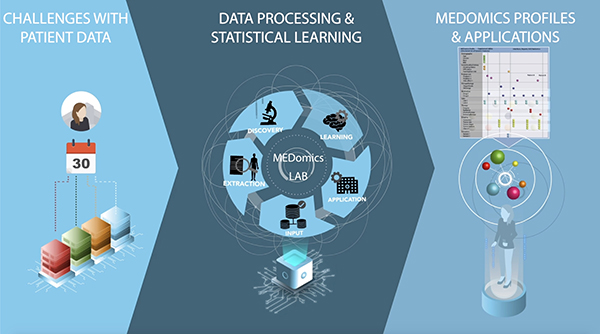
Despite widespread adoption of electronic health records (EHR), many hospitals are not ready to implement data science research in their clinical pipelines. With this in mind, the international MEDomics consortium has worked to create a secure, dynamic, continuously learning and expandable infrastructure, designed to constantly capture multimodal electronic health information, including imaging, across a large and multicentric healthcare system.
This project is led by Olivier Morin, PhD, UCSF ci2 member and interim chief of physics in the Department of Radiation Oncology. The result of three years of their work was recently published in Nature Cancer.
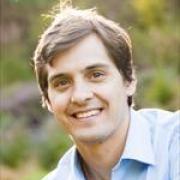
"We reported results of the first longitudinal approach to natural language processing (NLP) of unstructured medical notes and demonstrate its ability to update and improve a prognostic model over time, as a patient's oncologic illness course unfolds," says Dr. Morin.
The framework developed by the team of investigators is a continuously learning infrastructure through which multimodal health data are systematically organized and data quality is assessed with the goal of applying artificial intelligence (AI) for individual prognosis. The framework is currently composed of thousands of individuals with cancer and millions of data points over a decade of data recording. Their recent paper demonstrates prognostic utility of this framework in oncology.
This work was initiated at UCSF and it was done through international collaboration between UCSF, McGill and Université de Sherbrooke in Montreal, QC, Canada, OncoRay in Dresden, Germany and Maastricht University in the Netherlands.
As proof of concept, the investigators also reported an analysis using this infrastructure, which identified the Framingham risk score to be robustly associated with mortality among individuals with early-stage and advanced-stage cancer.
"With this data we were able to validate findings of published clinical trials using real world data, e.g. the positive impact of immunotherapy in lung cancer," says Catherine Park, MD, chair of UCSF Radiation Oncology and co-senior author. "In addition, there are exciting opportunities to generate hypothesis based on associations from patients' individual health profiles, and risk factors."
"Our vision is to create an open-source computation platform integrating all MEDomics developments and from which both clinical staff and research scientists could tackle a diverse range of oncological problems using AI," says Dr. Morin. He notes that part of the code is open source and they we would like to expand the international MEDomics consortium. Learn more.
Additional authors from the UCSF ci2 include Jorge Barrios Ginart, PhD, Taman Upadhaya, PhD, Gilmer Valdes, PhD and Javier Villanueva-Meyer, MD. Authors from UCSF Radiation Oncology include Steve Braunstein, MD PhD, William Chen, MD, Sue Yom, MD, PhD and Julian Hong, MD, MS.