A recent study from UC San Francisco and Florida Institute of Technology investigators used machine learning to predict the mortality and a few other investigated intermediate outcomes of neuroblastoma patients non-invasively from CT images.
"Neuroblastoma is one of the most common pediatric cancers," says Youngho Seo, PhD, UCSF Center for Intelligent Imaging (ci2) member and professor in the Department of Radiology and Biomedical Imaging. "In this study, we analyzed performances of multiple machine learning algorithms over retrospective CT images of 65 neuroblastoma patients."
Investigators used an artificial neural network (ANN) on tumor radiomic features extracted from 3D CT images. From there, a pre-trained 2D convolutional neural network (CNN) is used on slices of the same images. In addition, machine learning models are trained for various pathologically investigated outcomes of these patients. The manually segmented primary tumors were independently reviewed by a subspecialty-trained pediatric radiologist, and pyradiomics library is used to extract 105 radiomic features.
In this study, six machine learning algorithms were compared to predict the following outcomes:
- Mortality
- Presence or absence of metastases
- Neuroblastoma differentiation
- Mitosis-karyorrhexis index (MKI)
- Presence or absence of MYCN gene amplification
- Presence of image-defined risk factors (IDRF)
Prediction ranges over multiple experiments are measured using the area under the receiver operating characteristic (ROC-AUC) for comparison.
"Overall, our results show that the radiomics-based ANN method slightly outperforms the other algorithms in predicting all outcomes except classification of the grade of neuroblastic differentiation, for which the elastic regression model performed the best," says Dr. Seo.
Findings were recently published in the Journal of Digital Imaging, the official peer-reviewed journal of the Society for Imaging Informatics in Medicine (SIIM).
The full team of investigators include Mini Poon and Matthew Zapala, MD, PhD from UCSF Radiology; William Temple, MD, Kieuhoa Tran Vo, MD, MAS and Katherine Matthay, MD from UCSF Pediatrics and Gengbo Liu and Debasis Mitra from the Florida Institute of Technology.
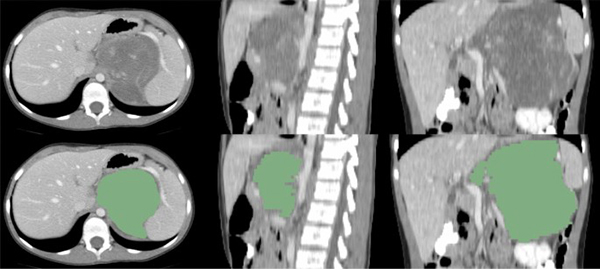