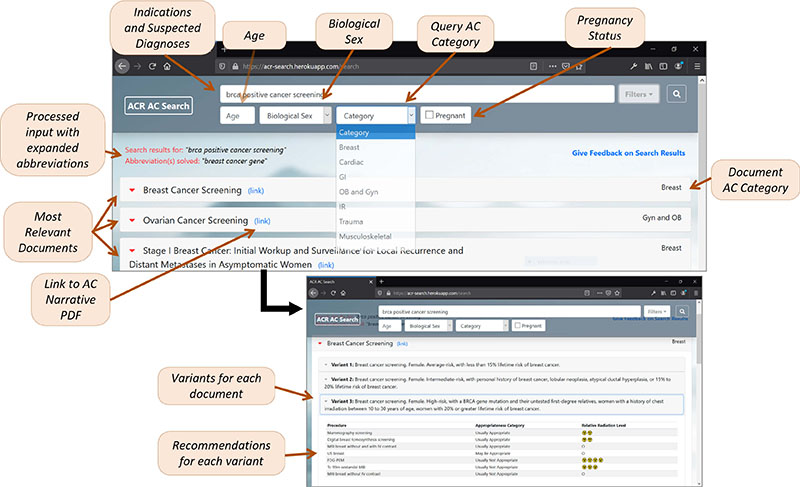
The ACR Appropriateness Criteria®(AC) are evidence-based guidelines to assist referring physicians and other providers in choosing the most appropriate imaging or treatment for a specific clinical condition. Employing these guidelines should help providers enhance quality of care and contribute to the most efficacious use of imaging. The comprehensiveness and maintenance of the AC makes it a unique resource for evidence-based clinical imaging decision support, but at this time, it's underused by providers and trainees.
In order to ease the use of these recommendations, the Big Data in Radiology (BDRAD) research team, co-founded by Jae Ho Sohn, MD, MS, physician-scientist and UCSF Radiology faculty and Center for Intelligent Imaging (ci2) member, developed and evaluated a natural language processing (NLP) search algorithm that automatically matches clinical indications that physicians write into imaging orders to appropriate AC imaging recommendations.
"Our approach can be integrated into imaging ordering systems for automated access to guidelines," says Dr. Sohn, corresponding author in this study.
To develop the algorithm, investigators applied a hybrid model of semantic similarity from a sent2vec model trained on 223 million scientific sentences, combined with term frequency inverse document frequency features. Imaging guideline documents were ranked based on their embeddings' cosine distance to query. Then, to test the algorithm, the team compiled a dataset of simulated simple and complex indications for each imaging guideline document (n = 410) and another with real-world clinical indications from randomly sampled radiology reports (n = 100). They compared their algorithm to a custom Google search engine.
"Our algorithm provides more relevant results than a custom Google search engine, especially for longer and more clinically complex imaging indications," shares Gunvant Chaudhari, the first author of the study and third year medical student at UCSF. Read more results in BMC Medical Imaging (PubMed).
The BDRAD research team works in close collaboration with many data scientists and computer scientists to understand how big data computational resources and computer vision advances can help problems in imaging sciences.
BDRAD investigators that were part of this study include Gunvant Chaudhari (MS3 student at the UCSF School of Medicine), Yeshwant Chillakuru (MS4 student at the George Washington University School of Medicine and Health Sciences), and Timothy Chen (incoming UCSF Radiology resident). UCSF Radiology and UCSF ci2 authors include Christopher Hess, MD, PhD, Valentina Pedoia, PhD, Youngho Seo, PhD and Thienkhai Vu, MD.