A multi-institutional group of researchers developed and tested a method to estimate and correct spatial distortions in functional magnetic resonance images (fMRI) without requiring the collection of additional scans. The team included Joseph (An Thanh) Vu, PhD, of UC San Francisco’s Center for Intelligent Imaging (ci2) and UCSF’s Salvatore (Sam) Torrisi, PhD. Colleagues from Vanderbilt University, Harvard University and the University of Sydney also contributed to the research.
The researchers share their conclusions in “Distortion correction of functional MRI without reverse phase encoding scans or field maps,” published in Magnetic Resonance Imaging by Elsevier. The first author is Tian Yu and the corresponding author is Kurt Schilling, both of Vanderbilt University.
fMRI data typically have spatial distortions which require correction. However, state-of-the art distortion correction methods require the collection of additional, time-consuming scans that can be difficult to implement and so are sometimes missing from study datasets altogether. The investigators developed a deep learning method called SynBOLD-DisCo (Synthetic BOLD contrast for Distortion Correction) to address this challenge.
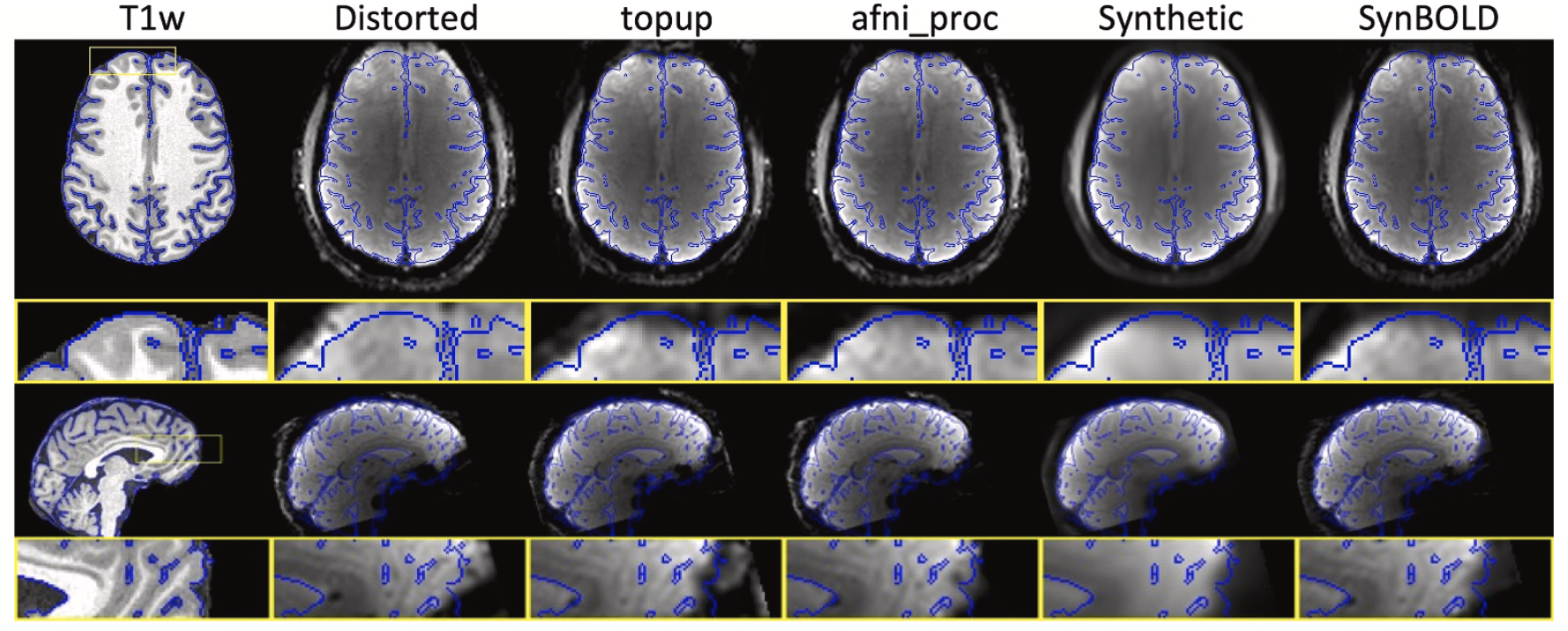
A T1w image is shown along with the distorted, topup-corrected, AFNI-corrected, synthetic, and final SynBOLD image. Edges from the structural image are overlaid on each to draw attention to areas of alignment/misalignment.
SynBOLD-DisCo combines T1-weighted anatomical images with BOLD fMRI images to synthesize “an undistorted image with contrast similar to the fMRI data” which can then be used as the “anatomical target for distortion correction,” writes Yu et al. The result yields fMRI data that are “virtually equivalent” to images corrected using standard methods.
SynBOLD-DisCo leverages deep learning to synthesize the images, relying on a conditional generative network that was trained, validated and tested on hundreds of datasets. The findings show that the method is highly effective at correcting fMRI distortions.
“Quantitative analysis suggests that the algorithm performs nearly as well as state-of-the art [methods] at correcting distortion artifacts in fMRI data,” writes Yu et al. “These methods … [show] consistent correction of observable distortions, and brain geometry more similar to the structural images than prior to correction.”
This method allows clinicians and researchers to collect high-quality fMRI data in less time, using fewer resources and to obtain more accurate results than without correction.
The investigators’ code and method are available as a Singularity container that can be integrated into current fMRI preprocessing pipelines. Learn more about the researchers’ methods in the article.
Additional co-authors of the article include Leon Cai, Victoria Morgan, Sarah Goodale, Karthik Ramadass, Dario Englot, Laurie Cutting, Catie Chang, John Gore and Bennett Landman of Vanderbilt University; Steven Meisler and Aaron Warren of Harvard University; and Jinglei Lv of the University of Sydney.
Read more about research and news at UCSF ci2.