There has been increasing interest in machine learning-based natural language processing (NLP) methods in radiology. However, at this time, models have often used word embeddings trained on general web corpora due to lack of a radiology-specific corpus. A team of investigators at the UCSF Center for Intelligent Imaging (ci2) set out to change that.
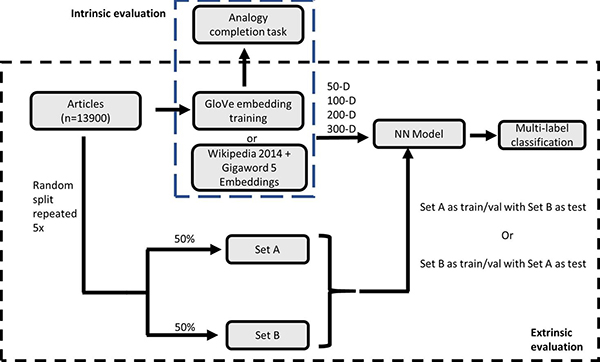
They examined the potential of Radiopaedia - a wiki-based international collaborative radiology educational web resource containing reference articles, radiology images and patient cases - to serve as a general radiology corpus to produce radiology specific word embeddings that could be used to enhance performance on an NLP task on radiological text. Lead author on this study was Timothy Chen, MS4 student at the University of Illinois College of Medicine. The original research was published in the Journal of Biomedical Informatics.
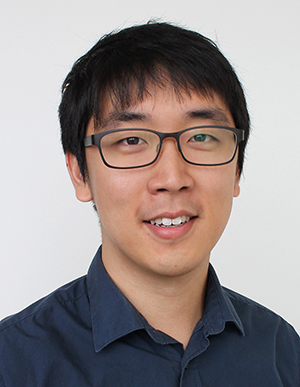
"The team reported a novel, radiology-specific word embedding that can help other radiological AI researchers conducting natural language processing projects. Overall, it can serve as a backbone in which other radiological language processing algorithms can be developed," says Jae Sohn, MD, MS, clinical fellow at UCSF Radiology, co-founder of the Big Data in Radiology (BDRAD) research team and a co-author on this research.
The BDRAD research team is part of the UCSF ci2, and it plays a key role in mentoring undergraduate and medical students who wish to learn about data science projects in radiology. The completely remote nature of the group has allowed students from around the world to join this team. Max Emerling (UC Berkeley), Gunvant Chaudhari (MS2 student at the UCSF School of Medicine) and Yeshwant Chillakuru (MS3 student at the George Washington University School of Medicine and Health Sciences) were also authors on this study. Youngho Seo, PhD and Thienkhai Vu, MD were UCSF Radiology and UCSF ci2 faculty mentors on this project.
"NLP is still a relatively unexplored tool in radiology," say the team of investigators. "We have presented one use case where word embedding pretraining on a radiology corpus is useful, but additional research is required to precisely identify the most effective scenarios for using radiology domain-specific word embeddings."
The source code, embeddings, and analogy dataset are publicly released. You can view the article in its entirety here.