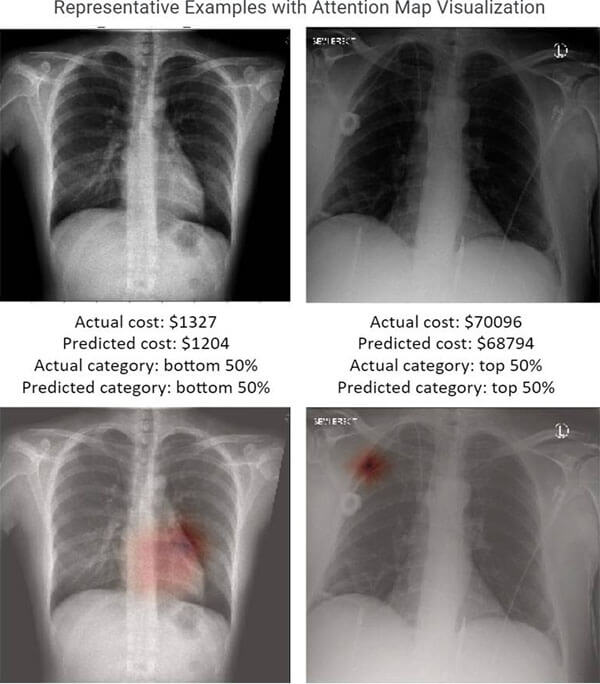
Health care cost is a barrier to health care access. Expenses are higher in sicker patients and those who are underserved in society. What can be done at both the individual patient level and the hospital and national healthcare policy level to help with cost estimation, accurate budget and planning for risk in reimbursement models based on health outcomes?
Artificial intelligence (AI) in medicine researchers have seen how recent advances in computer vision models, especially rapid advancements in convolutional neural networks (CNN), have contributed to a variety of applications. Deep learning has been especially power in identifying mild to moderate associations that humans might not routinely predict or detect in dense images.
Can AI tools be used to help health systems and payers to target care management and preventive health and develop cost and reimbursement plans? Researchers at the UCSF Center for Intelligent Imaging (ci2) set out to answer that question. In a recent pilot study, published in Scientific Reports, investigators hypothesized that chest radiographs (CXRs) capture many general health indicators so they may be used, potentially along with information on age, sex and ZIP code, to predict future medical costs.
“Our study aimed to help hospitals identify high-risk patients who will need expensive treatment, allowing healthcare organizations to map out care management plans and health and wellness plans. Additionally, this could help health systems and payers develop accurate budgeting models for reimbursement,” says Jae Ho Sohn, MD, MS, co-first author on this study along with Yixin Chen, PhD student at the Department of Computer Science, University of Illinois Urbana-Champaign.
A deep learning model was developed by retrospectively collecting 21,872 frontal CXRs from 19,524 patients with at least one-year spending data. Patients were non-obstetric adults who visited the emergency department and received a chest radiograph there or an outpatient facility on that same day. The best performing deep leaning models were able to relatively accurately identify which patients would have a top-50 percent personal healthcare cost after one, three, and five years with ROC-AUCs of 0.806, 0.771 and 0.729.
This study also demonstrates the potential of machine learning and AI technology in identifying hidden information underlying patient imaging such as patient health and socioeconomic risk factors that would otherwise not be evaluated in normal clinical radiology workflow. “Radiological imaging data is full of rich information that may not be routinely extracted by human radiologists, but big data and deep learning can,” says Youngho Seo, PhD, UCSF ci2 member and senior author on this study. “Physicians are trained to identify only a handful of imaging biomarkers known to medical literature and/or of direct relevance to clinical care, but our deep learning algorithm can analyze thousands of imaging features to identify weak to moderate correlations, such as future healthcare spendings.”
Thienkhai Vu, MD, UCSF ci2 member and UCSF chest radiology faculty was also a part of this study along with Jaewon Yang, PhD, current faculty at UT Southwestern. Additional contributors include Karen Ordovas, MD, former UCSF Radiology faculty and current chief of Cardiothoracic Imaging at the University of Washington; Dima Lituiev, PhD, senior machine learning scientist at the UCSF Bakar Computational Health Sciences Institute; Benjamin Franc, PhD, faculty in the Department of Radiology, Stanford University School of Medicine and Dexter Hadley, MD, PHD, MSE, chief of Artificial Intelligence at the University of Central Florida College of Medicine.