A team, including investigators from the UC San Francisco Center for Intelligent Imaging (UCSF ci2), reported on the development of a model that predicts the future tau burden in patients with Alzheimer's Disease (AD). The article, titled "Connectome-Mediated Prediction of Future Tau-PET Burden in Alzheimer's Disease," is published in the Journal of Biotechnology and Biomedicine.
Recent disease-modifying therapies for AD aim to stop or slow down tau aggregation, a biomarker preceding the appearance of AD symptoms. To evaluate the efficacy of these new AD drugs, scientists need an accurate way to predict how much tau a patient would have in the future. This would allow them to compare these predictions against measured values observed in clinical trials, essentially eliminating the need for expensive control arms. Developing accurate predictive models for the evolution of Alzheimer's disease is, therefore, of tremendous clinical importance.
In this work, the team followed 88 subjects who had been diagnosed with either Mild Cognitive Impairment (MCI) or AD and tracked their tau levels over 18 months. They used flortaucipir (FTP) positron emission tomography (PET) to measure the in vivo accumulation of tau. To predict the longitudinal changes of tau, the team incorporated the Network Diffusion Model (NDM) of tau-spread with data-driven approaches to "derive a computational biomarker of tau change that may be used as a monitoring and tracking tool in clinical trials."
By taking into account how neurons are interconnected in the brain and therefore how tau is expected to spread, the researchers were able to identify a small subset of six regions expected to receive the largest tau accumulation over the next 18 months. By focusing on this selected, high signal-to-noise group of regions, they found that the amount of tau accumulation could be predicted with extremely high accuracy.
"Our work has the potential to greatly strengthen the repertoire of analysis tools used in AD clinical trials, opening the door to future interventional trials with far fewer sample sizes than currently required," writes first author Pablo Damasceno, a former member of UCSF ci2.
They hope that, by providing a more accurate way to predict tau burden, pharmaceutical companies will be able to increase their confidence that they are seeing real effects of the drugs they are testing in clinical trials. This could help speed up the development of effective treatments for AD and ultimately improve the lives of those affected by this debilitating disease.
The investigators are associated with UCSF ci2, the UCSF Department of Radiology and Biomedical Imaging, the UCSF Bakar Computational Health Sciences Institute, the UCSF Memory and Aging Center, Eli Lilly and Company, Avid Radiopharmaceuticals, the Lawrence Berkeley National Laboratory and the Helen Wills Neuroscience Institute at the University of California, Berkeley. Additional authors from UCSF ci2 include senior author Ashish Raj, PhD, and Gil Rabinovici, MD, who are also UCSF Radiology faculty.
To read more about recent research and news from UCSF Ci2, visit our blog.
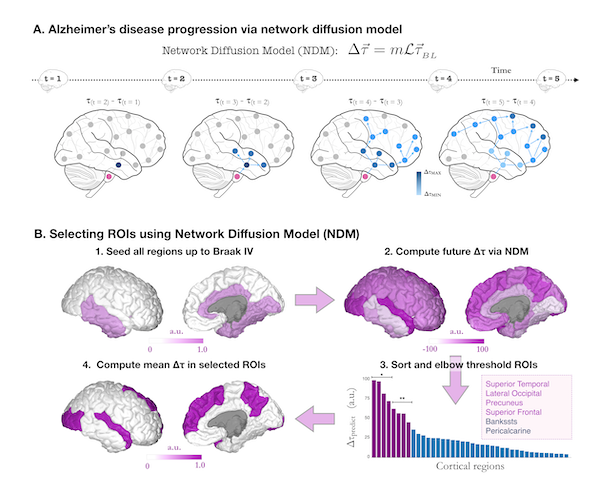