Principal author
Contributors
Valentina Pedoia, PhD, and Sharmila Majumdar, PhD, respectively an Assistant Professor and Professor in the Department of Radiology and Biomedical Imaging, are developing algorithms for advanced computer vision and deep learning for improving the usage of non-invasive imaging as diagnostic and prognostic tools of degenerative joint disease. Their lab has developed deep learning convolutional neural networks for musculoskeletal tissue segmentation, abnormality detection, and severity staging covering a diverse range of imaging modalities and diseases – including bone fractures, soft tissue degeneration, and sports injuries. This work has been supported by NIH R00 and R61 grants, as well as the Department’s ongoing partnership with GE Healthcare.
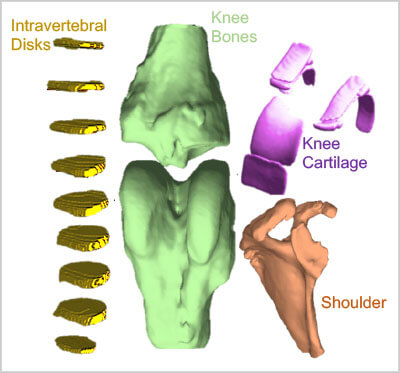
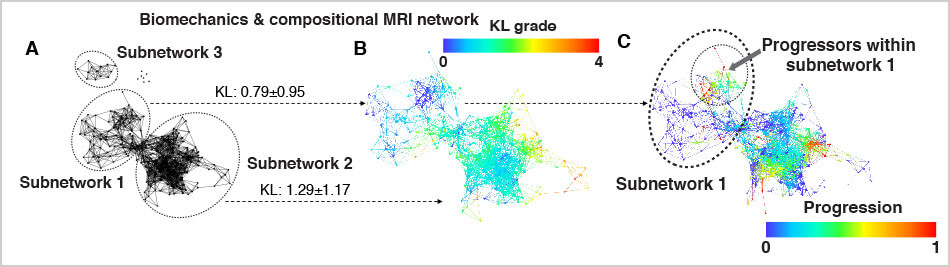