There is a growing interest in non-contrast breast MRI alternatives for tumor visualization to reduce the limitations associated with gadolinium administration. Maggie Chung, MD, UCSF Center for Intelligent Imaging (ci2) member and breast imaging clinical fellow in the Department of Radiology and Biomedical Imaging led a pilot study that demonstrated the feasibility of using deep learning to simulate contrast-enhanced breast MRI for imaging malignant masses.
“Recent brain MRI studies have shown that it’s feasible to synthesize contrast-enhanced images from pre-contrast inputs using deep learning. We aimed to apply a similar technique to breast MRI,” says Dr. Chung. “But there are several unique challenges with applying this technique to the breast compared to the brain including misregistration due to mobility of the breast tissue, greater field inhomogeneity, fewer pre-contrast series, and wider variability in tissue density and vascularity.”
With this in mind, the team of investigators set out to evaluate the feasibility and accuracy of generating simulated contrast-enhanced T1-weighted breast MRI from pre-contrast MRI sequences in patients with biopsy proven invasive breast cancer using deep learning.
“Our results found that it is feasible to generate simulated contrast-enhanced breast MRI using deep learning,” says Dr. Chung. “We found that both simulated and real contrast-enhanced MRI demonstrated comparable tumor sizes, areas of tumor enhancement, and image quality without significant qualitative or quantitative differences.”
The pilot study is currently in press in Radiology. Dr. Chung and team are looking forward to the release of the published paper which will share full methodology and detailed results.
This study demonstrated the feasibility of generating simulated contrast enhanced breast MRI with deep learning. Simulated contrast-enhanced MRI has the potential to extend the accessibility of breast MRI by reducing the need for contrast for tumor visualization. “As our next step, we look forward to evaluating the performance of simulated contrast in screening breast MRI.”
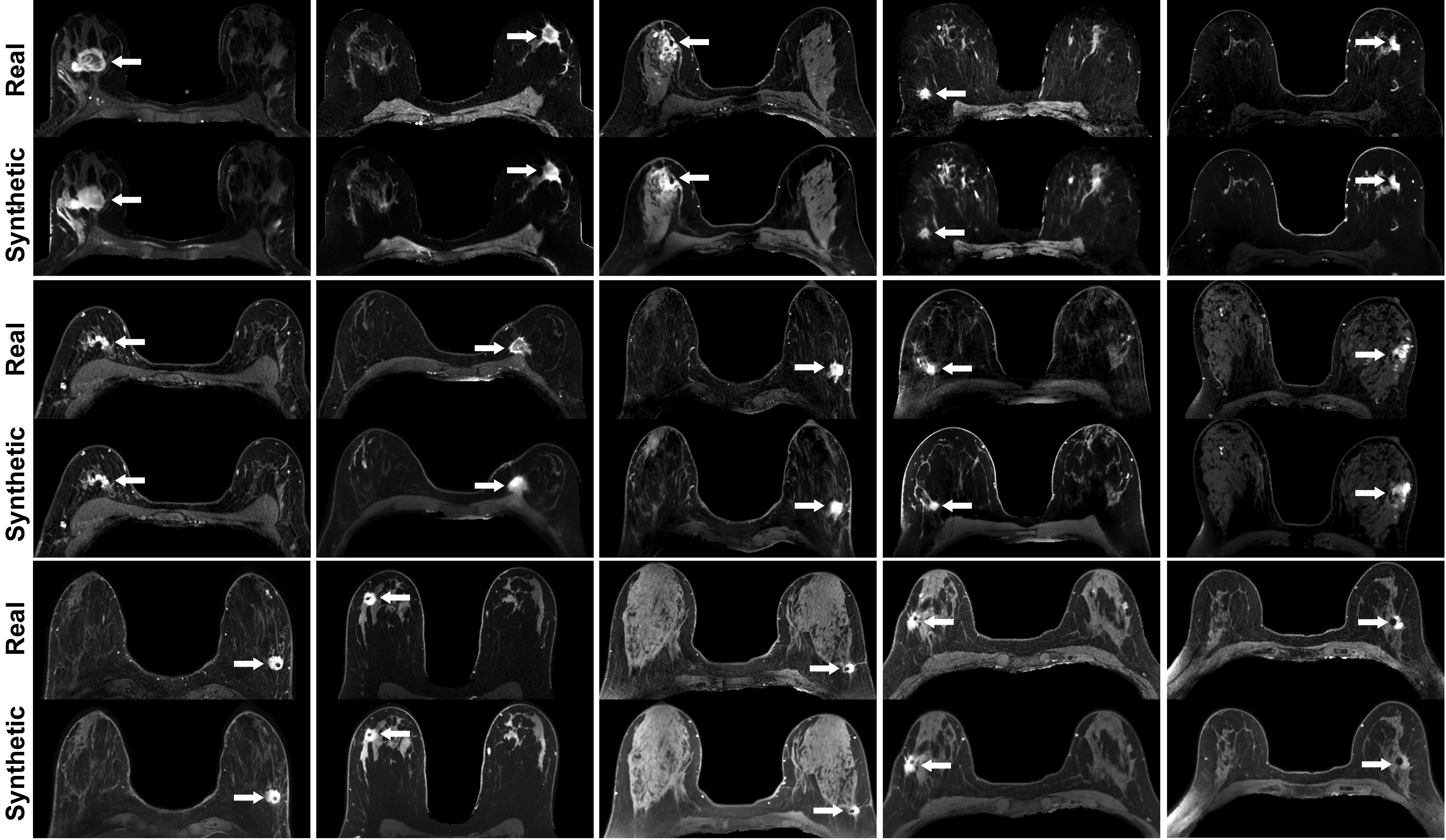