A team of researchers from UC San Francisco's Department of Radiology and Biomedical Imaging and Center for Intelligent Imaging (ci2) has developed a machine learning (ML) method for automating lumbar spine magnetic resonance imaging (MRI) acquisition.
The researchers discuss their work in "Machine learning-based automated scan prescription of lumbar spine MRI acquisitions," published in Magnetic Resonance Imaging journal. Eugene Ozhinsky, PhD, the lead author, is an assistant professor in the UCSF Department of Radiology and Biomedical Imaging (UCSF Imaging) and VA Advanced Imaging Research Center. His co-authors were Felix Liu, researcher, Valentina Pedoia, PhD, an associate professor at UCSF Imaging and Leader of Image Analysis at the Altos Labs, and Sharmila Majumdar, PhD, UCSF Imaging professor and Director of the Musculoskeletal Research Interest Group at UCSF. Drs Ozhinsky, Pedoia and Majumdar are ci2 members.
The authors write that planning MRI examinations is a manual process where an imaging technologist places a stack of slices over the low-resolution "localizer" images of a body part being examined. The quality of the images and their diagnostic value depends on the accuracy of their position and alignment with anatomical structures. The goal of the project was to improve the quality of MRI examinations by reducing prescription errors and enable more consistent and easier to interpret imaging studies.
To automate MRI scan planning, Dr. Ozhinsky and co-authors developed an ML-based software pipeline and installed it on a 3 Tesla MRI scanner. The pipeline was based on a novel Multislice Rotational Region-based Convolutional Neural Network (MS-R2CNN) architecture. Given access to previously-acquired images, the model could learn how trained operators positioned the slices and use that knowledge to prescribe new examinations.
The investigators used a dataset of 747 clinical lumbar spine MRI exams, each containing sagittal T1 and T2 weighted images and corresponding localizer images.
Researchers tested the software during MRI examinations of three healthy volunteers and twenty patients with lower-back pain. Their results showed that software was able to generate good prescriptions of the lumbar spine no matter how the volunteers were positioned inside the MRI and acquired high-quality images. In patients with lower-back pain, the generated prescription was applied in 18 cases (90% of the total number). None of the cases required major adjustment, while in 11 cases (55%) there were minor manual adjustments to the generated prescription.
The authors conclude that their study demonstrates the ability of oriented object detection-based models like MR-R2CNN to be trained to prescribe oblique lumbar spine MRI acquisitions and to do it directly on the scanner within the time constraints of a clinical MRI examination. They are working on extending this technique to other organs and acquisition types.
Read more about research and news at UCSF ci2.
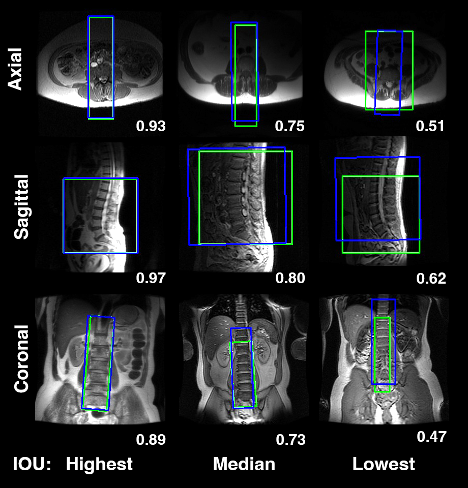
Oblique prescriptions of the lumbar spine MRI acquisitions, generated by MS-R2CNN network (blue) and ground truth prescriptions, performed by trained MRI technologists (green), overlaid on the localizer image slices along with the respective intersection over union (IOU) metrics.