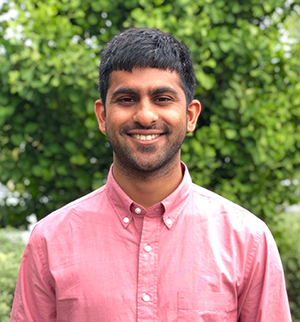
It's National Postdoc Appreciation Week, a time to recognize the significant contributions that postdoctoral scholars make to research and discovery. Here at the UCSF Center for Intelligent Imaging (ci2) postdoctoral scholars play a key role and work closely with our principal investigators (PIs). We caught up with Abhejit Rajagopal, PhD, postdoctoral scholar, and asked him a few questions about how he got into AI in medicine, projects he's working on and more!
Dr. Rajagopal is an electrical engineer by training, with a background in electromagnetics, signal processing, and numerical analysis. He began his scientific journey in and out labs at UC Irvine and UCLA, learning to fabricate, model, and image nanoscale transistors. While attending graduate school at UC Santa Barbara, he discovered the fascinating art of image reconstruction: (making pictures of objects from measured data) and have been applying neural networks and non-linear regressors to various imaging modalities ever since. After that, he came to UCSF.
"I joined UCSF for my postdoc to apply my deep learning techniques to the medical domain, finding applications in both enhanced imaging but also in enhanced cancer classification capabilities," says Dr. Rajagopal.
Tell us about the types of projects you are working on.
So many! There are three specific areas I've been developing at UCSF, and one long-term vision. Since I joined in 2019, I've been working in the PET/MRI space, trying to increase the resolution and consistency of next-generation PET imaging using machine learning (the subject of my NIH-F32 postdoc grant). Our team has achieved this by combining deep learning predictions (i.e., UNet) with physical measurements from the sensor, to strictly improve performance. The obvious clinical application of this work is enhanced image quality and quantification of disease!
Another project I've been working on with Kirti Magudia, MD,PhD (former UCSF Radiology clinical fellow) is using deep learning to enable enhanced prostate cancer classification from MRI. This has the potential to improve prostate MRI-based screening by providing predicted “cancer maps” to the clinician, which can be used for both image-based staging of disease as well as for biopsy and radiotherapy treatment - the subject of a recent pilot award I have with Jessica Scholey, MS (UCSF ci2 member and clinical instructor with UCSF Radiation Oncology).
The third project I’ve been working on is understanding and improving the generalization capabilities of neural networks. As deep learning increases in adoption in radiology and medicine as whole, my goal is to make sure clinicians know when—and why—they can trust AI-generated predictions. Our team has achieved this by analyzing the nodes of deep learning algorithms to quantify out-of-distribution predictions.
My vision for the next stage of my career is to utilize machine learning to build better, more affordable, point-of-care medical imaging technologies that leverage state of the art scanners only available in well-funded hospitals.
Which UCSF Radiology and UCSF ci2 PIs have you worked with?
I've been working primarily with Peder Larson, PhD and Thomas Hope, MD in the Advanced Imaging Technologies group. However, we love collaboration, so drop us a line if any of our work interests you!