Congenital heart disease (CHD) is the most common birth defect globally affecting about one percent of births per year worldwide, according to the Centers for Disease Control and Prevention (CDC). It can vary from mild to severe, and babies born with critical CHD will need surgery or other procedures in the first year of life. While fetal screening ultrasound provides five views of the heart that together can detect 90 percent of complex CHD, not all centers have the same detection rates. In practice, sensitivity can be as low as 30 percent. As such, there is a critical and global need to improve detection of fetal CHD within clinical guideline-recommended medical imaging.
"That's a surprising gap between the possible and the real world and it's not just explained by women not being able to get ultrasound," says Rima Arnaout, MD. As a cardiologist, Dr. Arnaout is a faculty member in the UCSF Department of Medicine (Cardiology), Biological and Medical Informatics (BMI) Graduate Program, Bakar Computational Health Sciences Institute (BCHSI) and Center for Intelligent Imaging (ci2). She studies how machine learning and artificial intelligence (AI) can reduce diagnostic errors in medical imaging and lead to new insights on cardiovascular disease.
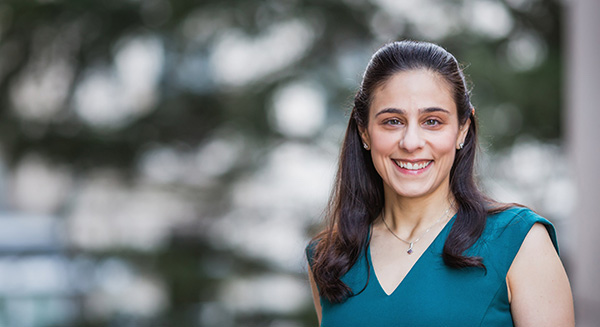
Recently, Dr. Arnaout, her lab and key collaborators used machine learning to successfully screen for fetal heart defects in one of the most widely used diagnostic tests – the second-trimester ultrasound. Their work was recently published in Nature Medicine.[1]
"We were able to combine clinical insights with an ensemble of neural networks to facilitate data-efficient strategies for improving clinically relevant use cases, even for rare diseases, and we can do this in a way that takes advantage of existing clinical guidelines rather than competing with them," said Dr. Arnaout when presenting this work at NVIDIA's GPU Technology Conference (GTC).[2]
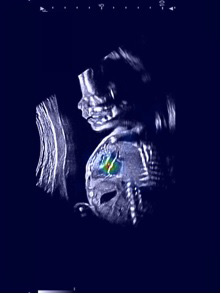
They utilized 107,823 images from 1,326 retrospective echocardiograms and screening ultrasounds from 18- to 24-week fetuses to train an ensemble of neural networks to identify recommended cardiac views and distinguish between normal hearts and complex CHD. In addition, they used segmentation models to calculate standard fetal cardiothoracic measurements.
The model achieved an area under the curve (AUC) of 0.99, 95 percent sensitivity (95 percent confidence interval (CI), 84–99 percent), 96 percent specificity (95 percent CI, 95–97 percent) and 100 percent negative predictive value in distinguishing normal from abnormal hearts on a test set of over four thousand screening fetal ultrasounds (over 4.4 million images). This performance represents a great improvement over the 30-50 percent sensitivity found in some screening centers worldwide and rivals expert screening.
This is significant because generally, AI models are trained with 90 percent of available data and the remaining 10 percent serve as the test cases. The researchers flipped that approach and tested the model on 400 times the number of ultrasound images than it had been trained on, in order to demonstrate model generalizability.
[1] Dr. Arnaout was corresponding author on this study. Additional authors include Erin Chinn, MS and Lara Curran, MBBS BSc, members of the Arnaout Lab; Anita Moon-Grady, MD, director of the Fetal Cardiovascular Program at UCSF and collaborator with the Arnaout Lab and Jami Levine, MD, attending physician at Boston Children's Hospital and assistant professor of pediatrics at Harvard Medical School.
[2] UCSF ci2 researchers collaborate with NVIDIA developers on AI tools for clinical radiology using NVIDIA Clara and NVIDIA DGX-2.