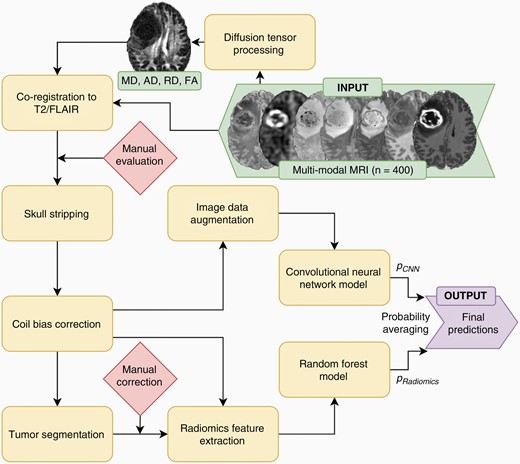
Glioblastoma is the most common primary brain tumor, but it remains difficult to treat despite advances in surgical, radiation, and medical therapies. Individualized tumor genetic assessment has become important for accurate prognosis and for guiding emerging targeted therapies, yet challenges remain for widespread tumor genetic testing due to costs and the need for tissue sampling.
Investigators at the Center for Intelligent Imaging (ci2) and the Departments of Radiology and Biomedical Imaging, Neurology and Neurological Surgery and Pathology at UCSF set out to evaluate a novel artificial intelligence (AI) method for non-invasively predicting clinically relevant genetic biomarkers from preoperative brain MRI in patients with glioblastoma. Study findings were recently published in Neuro-Oncology Advances, a journal for the Society for NeuroOncology (SNO).
“We developed models to predict biomarker status from MRI data using radiomics features, convolutional neural network (CNN) features, and a combination of both and assessed 9 different genetic biomarkers that are relevant for treatment or prognosis,” says Evan Calabrese, MD, PhD, neuroradiology clinical fellow at UCSF and corresponding author on this study. “We determined that combining radiomics and convolutional image features yielded improved performance.”
According to investigators, accurate radiogenomic assessment of preoperative brain MRI has potential to improve care of patients with glioblastoma, and this could be particularly useful for helping to guide the use of emerging targeted therapies.
“This study is important because it demonstrates accurate preoperative radiogenomic assessment of several common glioblastoma genetic biomarkers including some that are relevant to investigational therapies,” says Dr. Calabrese. “Additionally, we further demonstrate that combining information from radiomics and deep learning feature extraction methods can lead to improved radiogenomic prediction accuracy compared to using either type of feature alone.”
This approach presented in this study has shown promise for other radiologic/oncologic inference tasks, and it may ultimately prove to be an important step toward integration of image-based genetic inference into clinical workflows. What’s next? According to investigators, future work will be necessary to evaluate the optimal architectures for combining radiomics and CNN features and for assessing generalizability and portability to other datasets.
This team of investigators included Andreas Rauschecker, MD, PhD and Javier Villanueva-Meyer, MD, UCSF ci2 members and UCSF Radiology faculty; Soonmee Cha, MD, UCSF Radiology and UCSF Neurological Surgery faculty; Jennifer Clark, MD, MPH, UCSF Neurology and Neurological Surgery faculty; David Solomon, MD, PhD, neuropathologist and UCSF Pathology faculty and Jeff Rudie, neuroradiology at Scripps Clinic and adjunct faculty at UC San Diego.