As an emerging hybrid technology PET/MRI brings together the strength of functional and molecular contrast from PET with the soft-tissue and structural contrast from MRI scans by simultaneously acquire both datasets to capture a wealth of diagnostic information in a single scan while also using less radiation. PET/MRI technology is available for clinical use today at institutions like UCSF, and scientists continue to work on developing imaging reconstruction methods both for neurological, oncological, and whole-body applications of this cutting-edge technology.
Here at the UCSF Center for Intelligent Imaging (ci2), a team of investigators led by Peder Larson, PhD and Thomas Hope, MD are currently working on improving simultaneous PET/MR systems using machine learning. The team has published 14 papers on improving these systems– so far!
1. Using deep learning for PET/MRI attenuation correction
Accurate quantification of uptake on PET images depends on accurate attenuation correction in reconstruction. However, this information is difficult to measure with MRI, and this challenge has been a major factor limiting more widespread adoption of PET/MR.
The team has developed several generations of MR-based attenuation correction methods, both for body and head applications. These are based on fat and water maps derived from a 2-echo Dixon MRI sequences, specialized ultrashort-echo-time or zero-echo-time (ZTE) pulse sequences that can capture bone information, and MRI-to-CT image translation methods based on deep learning.
In this project, the team developed, trained and tested "ZTE and Dixon deep pseudo-CT (ZeDD CT)" a deep learning model using patient-specific multiparametric MRI consisting of Dixon MRI and proton-density-weighted ZTE MRI to directly synthesize pseudo-CT images that are used for PET attenuation correction. Overall, they found that their model produced natural-looking and quantitatively accurate pseudo-CT images and reduces error in pelvic PET/MRI attenuation correction compared with standard methods. In subsequent work, the team set out to investigate how the selection of MRI inputs affect the resulting output using a fixed network. They found that Dixon MRI alone may be sufficient for quantitatively accurate pseudo-CT images in the body. The lead author on this work was Andrew Leynes, a UCSF Bioengineering graduate student researcher and graduate of UCSF Masters of Biomedical Imaging program, and it was published in the Journal of Nuclear Medicine.[1] and at the 2018 IEEE International Conference on Acoustics, Speech and Signal Processing (ICASSP).
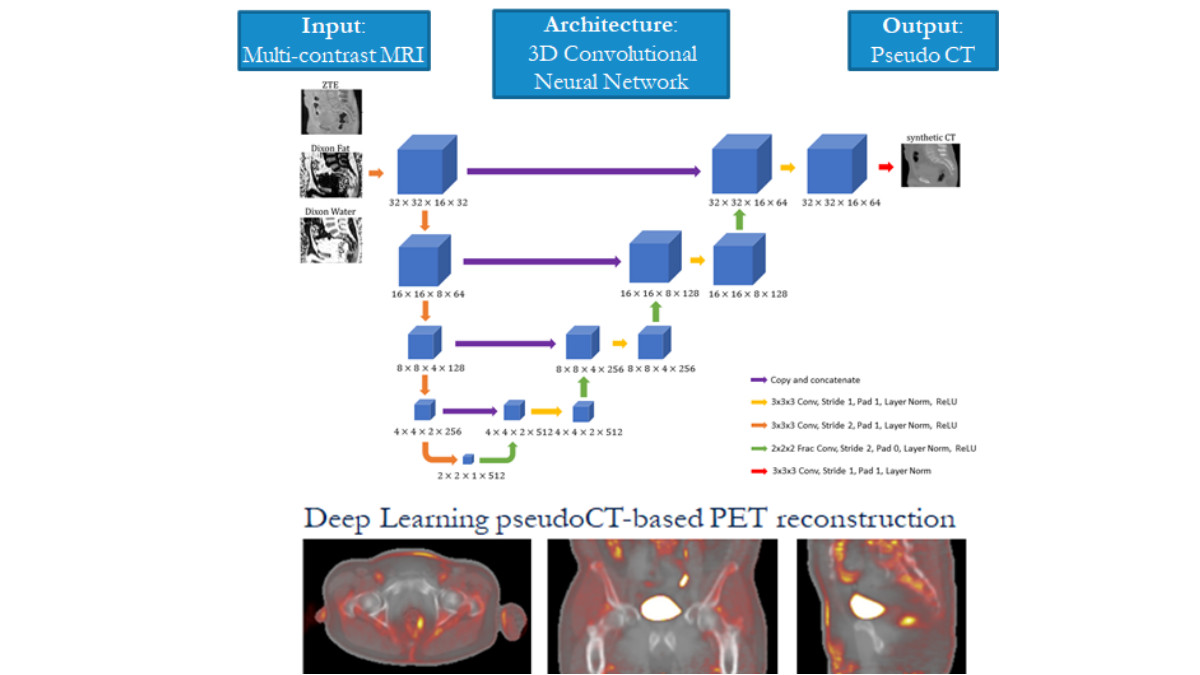
2. Incorporating high resolution structural information from MRI into the reconstruction of PET imagery
Most recently, the team presented a new strategy for incorporating high resolution structural information from MRI into the reconstruction of PET imagery via deep domain translated image priors using a two-step process. "The key idea of our approach is that domain translated PET imagery can capture the true spatial and sparsity patterns of PET imagery, which can be used to guide the convergence of the statistics-limited inverse problem," say the UCSF investigators - Abhejit Rajagopal, PhD and Nicholas Dwork, PhD (postdoctoral scholars) with Drs. Larson and Hope.
"This scheme can be superior to joint-sparsity reconstruction, among other methods, since the mismatch between PET and MRI features is significantly reduced by using the domain translated zero-dose PET as the prior instead. We evaluate this technique on a whole-body Fludeoxyglucose (18F) (18F-FDG-PET) dataset, demonstrating that dichromatic interpolation can recover high quality PET imagery from noisy and low dose PET/MRI, with no observed failure cases," they continue. The work can be seen online via the Society of Photo-Optical Instrumentation Engineers (SPIE).
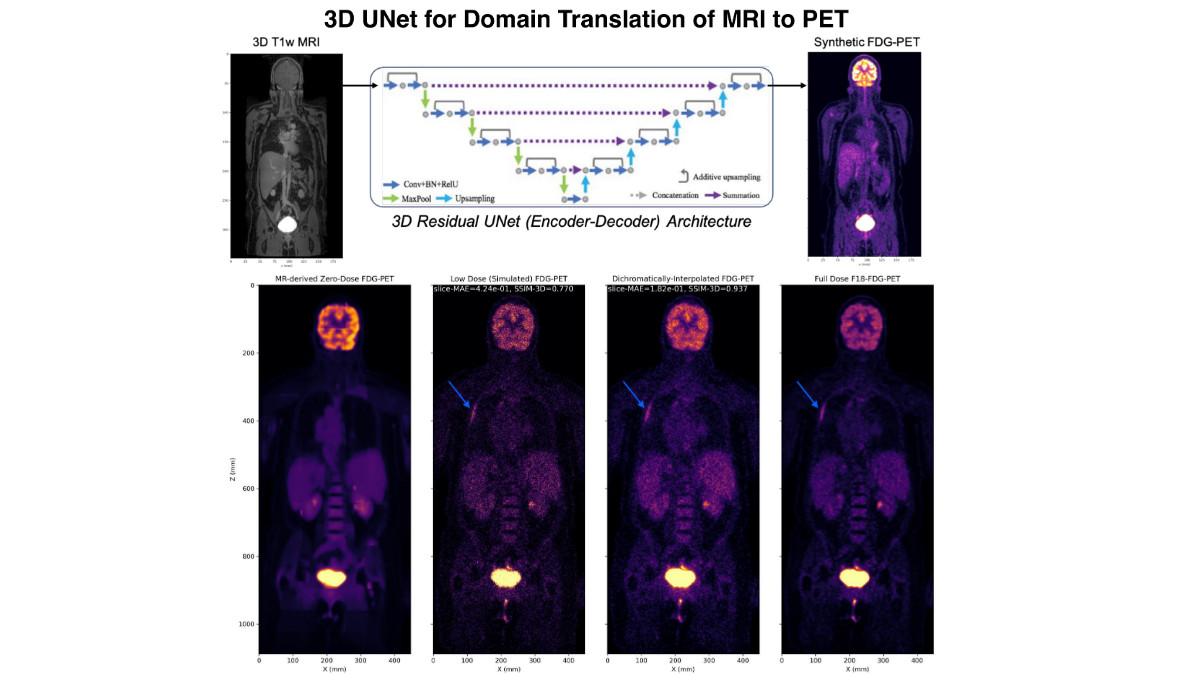
Overall, continued improvements to PET/MRI can benefit both research and clinical applications of these powerful systems. We'll continue to follow the work of UCSF ci2 investigators on this project.
[1] The full list of authors includes Jaewon Yang, PhD, associate specialist at UCSF Radiology; Thomas Hope, MD, Peder Larson, PhD and Youngho Seo, PhD, UCSF ci2 members and UCSF Radiology faculty, and Florian Wiesinger, Sandeep Kaushik, and Dattesh Shanbhag from GE Global Research.