Multi-Institutional Team Develops Deep Learning Technique for Generating Synthetic PET Sinograms from MRI
A multi-institutional team, including researchers from UC San Francisco’s Center for Intelligent Imaging (ci2), developed a deep learning technique that generates positron emissions tomography (PET) sinograms from whole-body magnetic resonance imaging (MRI).
The researchers share their conclusions in "Synthetic PET via Domain Translation of 3-D MRI,” published in IEEE Transactions on Radiation and Plasma Medical Sciences. Abhejit Rajagopal, PhD, the co-director of education and internships at ci2, is the first author. Thomas Hope, MD, the Vice Chair of Clinical Operations and Strategy in the UCSF Department of Radiology, is the corresponding author; and ci2’s Peder Larson, PhD, is a co-author.
The authors demonstrate the ability to generate “synthetic but realistic whole-body PET sinograms from abundantly available whole-body MRI.” They trained the model without needing to acquire hundreds of patient exams.
“These results together show that the proposed [synthetic PET] sPET data pipeline can be reasonably used for development, evaluation and validation of PET/MRI reconstruction methods,” writes Dr. Rajagopal and co-authors.
“Synthetic medical imaging datasets are also gaining popularity for several emerging use cases,” added Prof. Larson, “such as creating large, realistic datasets without personal information to preserve privacy. This technique could also be used as a teaching or discovery tool since it will generate PET images that we expect to have normal distribution of the tracer, in other words PET images without disease, and then compared against cases with potential disease.”
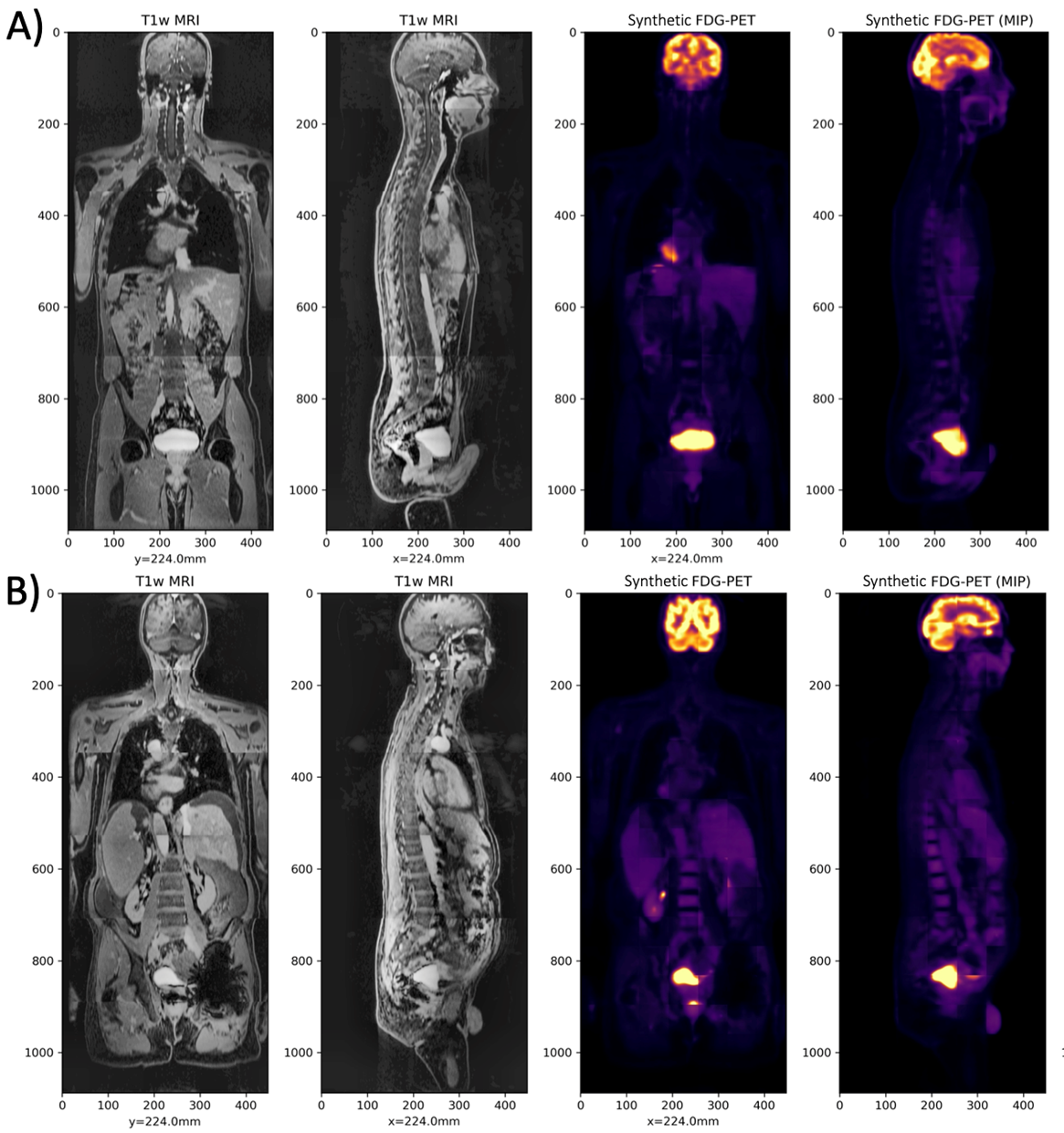
The left images are the T1-weighted MRI, and the right images are the Synthetic FDG-PET, for two cases in the test set.
The investigators used a dataset of 56 18F-FDG-PET/MRI exams to train the deep learning model. Validation of the model showed that the sPET data is interchangeable with real PET data and can be used with vendor-provided PET reconstruction algorithms like CT-based attenuation correction (CTAC) and MR-based attenuation correction (MRAC) to evaluate these techniques.
“We demonstrated its equivalent performance to real PET data for comparing CTAC and MRAC for PET reconstruction, and believe this result combined with the apparent realism of the synthetic images will make this method broadly applicable for evaluating the robustness of PET/MRI reconstructions and component techniques, including attenuation correction, scatter correction and MR-guided reconstruction algorithms,” writes Dr. Rajagopal and co-authors .
The source code for the model — include sPET training code, quantification experiments and more — is available for free on GitLab. Learn more about accessing and using the data in the article.
Additional co-authors of the article include:
- Yutaka Natsuaki of the University of New Mexico;
- Kristen Wangerin of G.E. Healthcare;
- Mahdjoub Hamdi, Hongyu An and Richard Laforest of Washington University in St. Louis;
- John Sunderland of the University of Iowa;
- and Paul Kinahan of the University of Washington.
Read more about research and news at UCSF ci2.