What better way to celebrate heart month than by sparing cardiologists from one of their more tedious tasks?
While deep machine learning has potential to improve workflows, diagnosis and measurement for cardiac ultrasound, deep learning models to date have relied on painstaking, manually traced heart chambers as training labels. Rather than saving clinicians work, deep learning can actually add to it by requiring these manual labels. Techniques to automatically label images that work well for high resolution imaging fail in the noisy, but clinically important, ultrasound modality.
However, new work from a team including researchers from the UC San Francisco Center for Intelligent Imaging (UCSF ci2) makes self-supervised segmentation of heart chambers possible in ultrasound, freeing humans from this extremely laborious task. Their work cuts down on the critical — but tedious and poorly reproducible — process of segmentation and measurement of cardiac chambers from ultrasound.
The preprint of the research, titled "Label-free segmentation from cardiac ultrasound using self-supervised learning," is available now on arXiv. The research was completed by members of the Arnaout Lab, including first author Danielle Ferreira, PhD, Zaynaf Salaymang, RDCS, and senior author Rima Arnaout, MD, of UCSF ci2.
The team trained the pipeline for self-supervised segmentation on echocardiograms from 450 patients and tested it on imaging from over 18,000 patients, including about half from an external center. Several clinical measurements were calculated from the model-derived segmentations and compared to real-world clinical measurements, finding the model to be similar.
While work is ongoing to improve even further, the results demonstrate a "human-label-free, valid and scalable" method for chamber segmentation from cardiac ultrasound. For example, investigators estimate that it would have taken a human about 2,500 hours to label the training data alone.
"Self-supervised segmentation of ultrasound represents a paradigm shift in how, rather than laboring to provide labels for data-hungry machine learning models, we can get machine learning to work for us efficiently, robustly and scalably, to solve important problems in cardiology and beyond," write the investigators.
Achieving label-free segmentation in ultrasound required the talents of a multidisciplinary team. "In achieving self-supervised segmentation for echocardiograms, we demonstrate how traditional computer vision techniques, deep learning, and clinical domain knowledge on chamber shapes and sizes can be combined for medical imaging tasks," writes the team.
Dr. Rima Arnaout is an Assistant Professor in Medicine (Cardiology) and a member of the Bakar Computational Health Sciences Institute, the Biological and Medical Informatics graduate program, the UCSF UC Berkeley Joint Program for Computational Precision Health, and Ci2. Dr. Ferreira is a data scientist at the Arnaout Lab. Ms. Salaymang is a practicing cardiac sonographer.
Read more about research and news at UCSF Ci2.
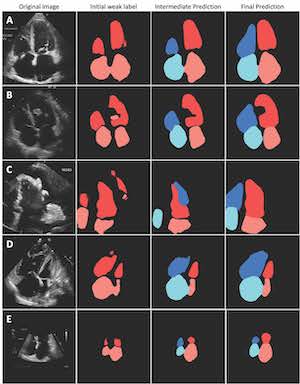