Hybrid PET/MRI systems combine the functional information from PET tracers with the soft tissue contrast from MRI. Here at the UCSF Center for Intelligent Imaging (ci2), Peder Larson, PhD and Thomas Hope, MD lead a team of investigators interested in developing improvements for simultaneous PET/MR systems using tools such as machine learning.
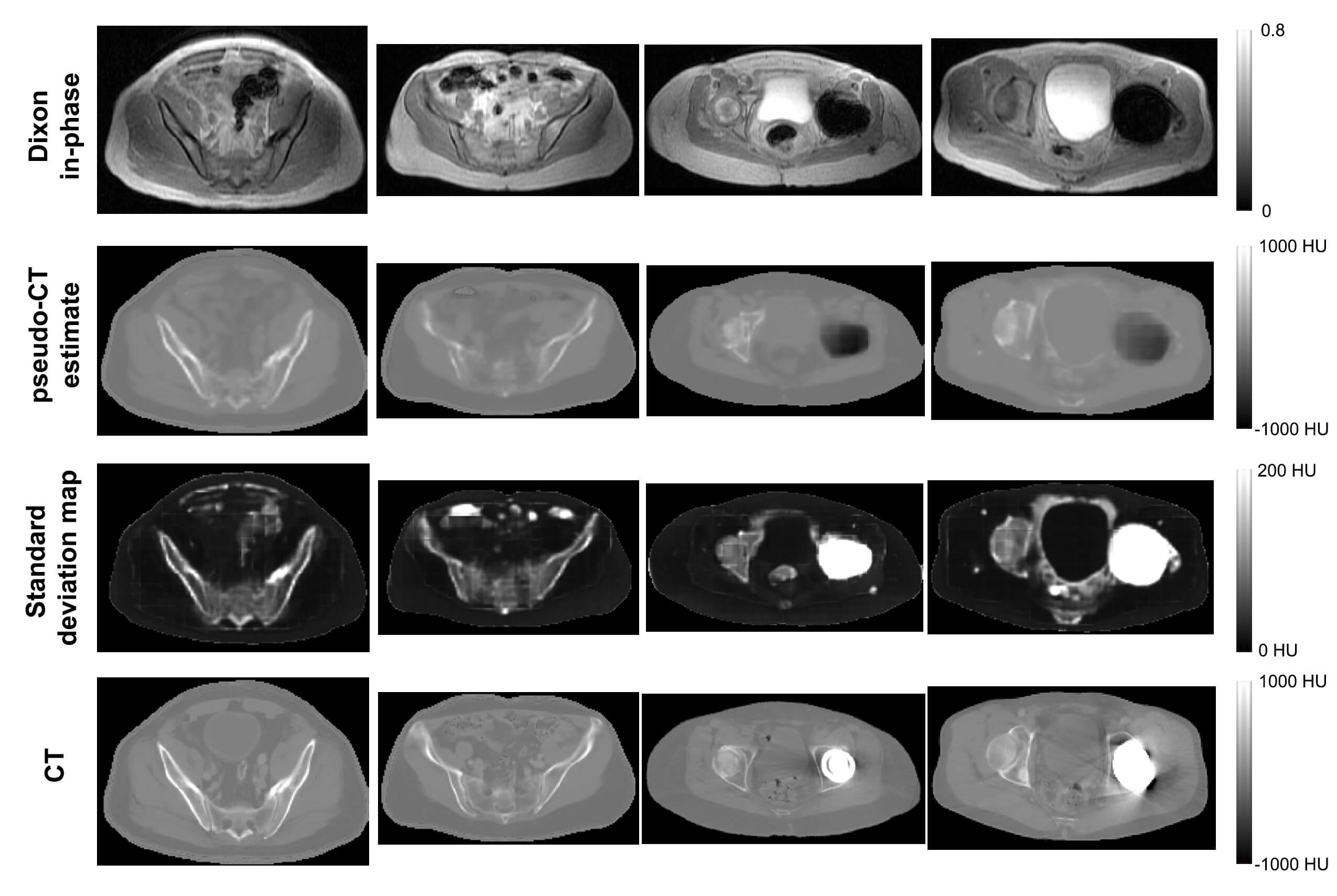
Last year, we shared a few highlights of the team's work on using deep learning for attenuation correction and incorporating high-resolution MRI data into the PET reconstruction. Most recently, the team, led by Andrew Leynes, a bioengineering graduate student in Dr. Larson's group, proposed a novel PET reconstruction method for PET/MR that leverages Bayesian deep learning and synergistically combines both MR and PET data. The first part of the method uses a Bayesian deep convolutional neural network to generate attenuation maps that are required for PET reconstruction from MR data. However, unlike conventional neural networks, the Bayesian CNN also produces uncertainty estimates to quantify the limitations of the attenuation maps. This is extremely valuable when the MR data is unreliable, for example due to motion, implants, and other imaging artifacts, as well as cases that are not captured in the training data. The attenuation maps and their uncertainty estimates are combined with the time-of-flight PET emission data in a maximum-likelihood estimation of activity and attenuation (MLAA) reconstruction for a truly synergistic PET/MR reconstruction.
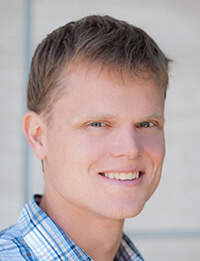
"A major remaining challenge for MR-based attenuation correction (MRAC) methods required for PET/MR is their susceptibility to sources of MRI artifacts and uncertainties due to the limitations of MRI contrast," explains Dr. Larson. "While the development of machine learning based methods have been a major advance for MRAC, they are also limited by these artifacts, uncertainties, and the range of anatomy captured in the training data. The proposed approach (UpCT-MLAA) created by Andrew Leynes cleverly combines Bayesian deep learning with MLAA to address all of these issues and demonstrated accurate estimation of PET uptake in pelvic lesions with recovery of signals near metal implants."
An early access version of this work was published in IEEE Transactions on Radiation and Plasma Medical Sciences. In addition to the UCSF investigators, Sangtae Ahn, Kristen Wangerin, Sandeep Kaushik and Florian Wiesinger from GE Healthcare, a UCSF ci2 industry partner, were also an integral part of this collaborative work.
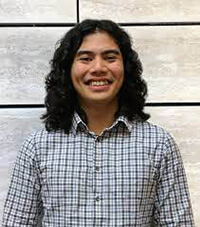
In patients without implants, the team found that UpCT-MLAA performed comparably to the state-of-the-art Zero-echo-time and Dixon Deep (ZeDD) pseudo-CT method (also created by Mr. Leynes). In patients with metal implants, however, all prior methods (ZeDD, other pseudo-CT methods, MLAA) either failed or had artifacts, but the UpCT-MLAA method was able to recover the high attenuation of the metal implant with accurate depiction of the rest of the volume.
"Attenuation coefficients from prior methods can either be accurate in normal anatomy but with the metal implant region having a very low attenuation coefficients (in actuality implants have extremely high attenuation) or be accurate in the metal implant region but having normal anatomy obscured by noise and artifacts. UpCT-MLAA properly estimated attenuation coefficients of metal implants as well as accurate anatomic depictions outside of implant regions," said Andrew Leynes.
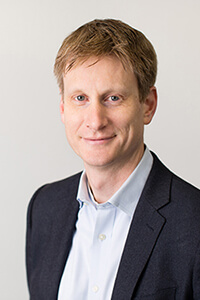
Dr. Larson notes how the Bayesian deep learning approach enabled identification of other limitations of the MR data beyond implants including high uncertainty in bone and bowel air, MRI artifacts such as motion and edge of FOV arm truncation, and dataset mismatch between training data and actual scans (e.g. arms up vs down). According to Dr. Hope, this will enable more patients, such as those with implants, to benefit from a simultaneous PET/MR scan, and will provide radiologists and their clinical colleagues with more accurate PET measurements to assess disease and monitor response to therapy.
Overall, continued improvements to this emerging PET/MRI hybrid technology will benefit both research and clinical applications of these powerful systems. We'll continue to follow the work of these UCSF ci2 investigators on this project.
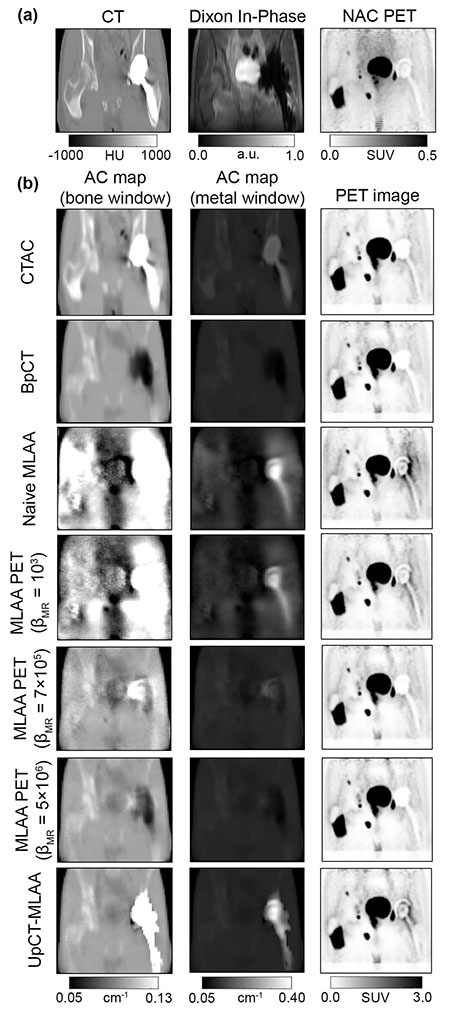