Here at UC San Francisco, Jessica Scholey, PhD, MSc focuses on radiation oncology applications of MRI, proton therapy and deep learning for medical imaging. As a member of both the Center for Intelligent Imaging (ci2) and the Department of Radiation Oncology faculty, Dr. Scholey's research includes the application of MRI technology to radiation oncology including MRI-only treatment planning of photon and proton radiotherapy.
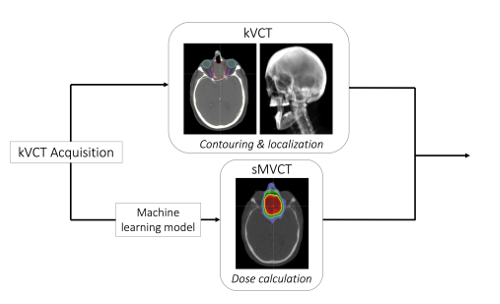
"In addition to MRI simulation for radiotherapy, our group focuses on using deep learning in medical imaging to improve dose calculation accuracy through more accurate estimations of electron density (for photon radiotherapy) and stopping power ratio (for proton radiotherapy)," says Dr. Scholey.
Dr. Scholey recently teamed up with fellow UCSF ci2 members Martina Descovich, PhD and Peder Larson, PhD; Sue Yom, MD, PhD, MAS, FASTRO, UCSF Radiation Oncology faculty; Atchar Sudhyadhom, PhD, assistant professor at the Brigham and Women's Hospital Department of Radiation Oncology, Vasant Kearney, CTO at Retrace and Luciano Vinas, from the UC Berkely Department of Physics to work on improving the accuracy of relative electron density and proton stopping power ratio through CycleGAN machine learning.
Their paper was recently published in Physics in Medicine & Biology, a journal published by IOP Publishing on behalf of the Institute of Physics and Engineering in Medicine.
According to the team of investigators, kilovoltage computed tomography (kVCT) is the cornerstone of radiotherapy treatment planning for delineating tissues and towards dose calculation. While kVCT provides excellent image contrast and signal-to-noise ratio, and it may have greater uncertainty in determining relative electron density and proton stopping power ratio (SPR). Conversely, megavoltage CT (MVCT) may result in superior dose calculation accuracy due to the underlying physics of how photons interact at megavoltage (MV) versus kilovoltage (kV) energies. "For this study, we wanted to convert kVCT HU to MVCT HU using deep learning to obtain higher accuracy electron density and SPR," explains Dr. Scholey.
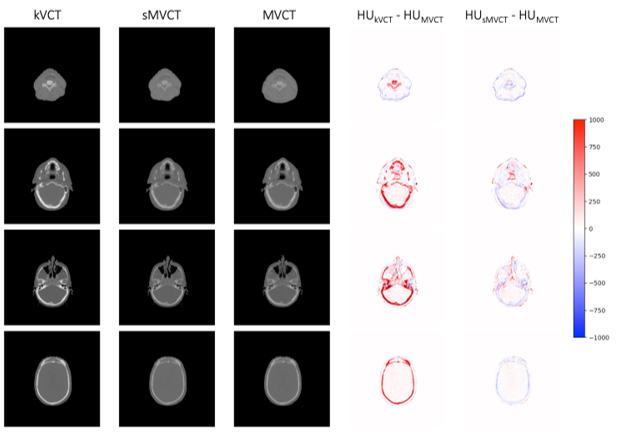
The approach taken by the team was to create tissue-mimicking phantoms used to compare kVCT- and MVCT-determined electron density and SPR to physical measurements. A deep learning model was trained using 100 head-and-neck datasets to learn the relationship between kVCTs and MVCTs and create synthetic MVCTs (sMVCTs). Read the research abstract for a full list of results.
"Our results showed that deep learning can be used to map kVCT to sMVCT, suggesting higher accuracy electron density and SPR is achievable with sMVCT versus kVCT," says Dr. Scholey. "In this workflow, we propose that the original kVCT images, with the high contrast and low noise intrinsic to kV imaging, be leveraged for localization and contouring of normal and diseased tissues while the sMVCT generated from the machine learning model be used for dose calculation (as shown in Figure 1). We are also expanding similar deep learning techniques for MRI."