Inter-reader disagreement due to differences in opinions among medical experts is a persistent problem across medical disciplines, including diagnostic radiology. Interpreting complex lesions on MRIs is no different and can result in varying findings even among experienced radiologists at the same level of expertise. How do we then improve consensus among radiologists, which can also lead to more accurate diagnoses? Is there a way to leverage collective intelligence of multiple experts without the social and cultural biases observed in group settings?
Rutwik Shah, MD, project director, and colleagues at the UCSF Center for Intelligent Imaging (ci2) answered these questions in a recent paper, in preprint at time of publication. They explored the use of swarm intelligence to address the two problems simultaneously:
1. Low consensus among experts.
2. Interpersonal biases seen in teams-based decisions
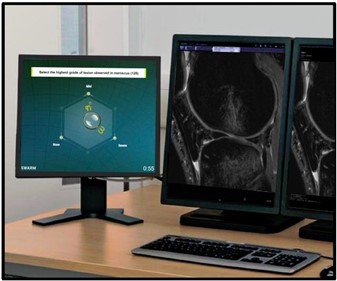
In a research collaboration with Unanimous AI, ci2 investigators leveraged the proprietary digital collaboration platform called Swarm®. According to Gregg Willcox, director of research and development (R&D) Unanimous AI, this technology, modeled after swarms in nature, "amplifies the intelligence of human groups, allowing them to make more accurate forecasts when swarming than when voting or acting as individuals. It does this by facilitating an anonymous, real-time, nonverbal deliberation between participants that combines the knowledge, wisdom, and insights of each group member into a single collective sentiment."
The study had participation of two separate groups of radiologists at different levels of expertise: 1) three board certified MSK radiologists (attendings) and 2) five radiology residents (trainees). Each group collaborated on the digital swarm platform (see Figure 1) in real time and anonymously, while simultaneously assessing 36 knee MRIs for meniscus lesions on PACS.
In each of the two groups, the swarm consensus outperformed the individual diagnoses, the post-hoc majority vote and the most confident vote. The attending swarm consensus saw a 23% improvement in agreement with ground truth and the resident swarm consensus saw up to a 30% improvement in agreement with ground truth. Moreover, the improvement observed with a 5-resident swarm was higher than a three-resident swarm, suggesting a positive effect of participant number.
Additionally, the team also compared the inference results from a state-of-the-art artificial intelligence (AI) system1 on the same set of 36 knee MRIs, trained to detect meniscus lesions. Although its accuracy was in line with individual radiologists, the swarm consensus significantly outperformed these results as well.
The use of Swarm platform has potential applications both for clinical use and research. Clinically, the platform can be utilized in cases with low consensus which require a follow-up scan or arbitration read. Given its easy access via a web browser, it also allows real-time collaboration between radiologists separated by geographical distance.
In research, swarm consensus can be used for better data labeling to train robust AI algorithms. As demonstrated, present AI solutions perform at best as individual radiologists. To improve results further, swarm provides an alternate approach to current strategies of using larger training datasets or more compute, neither of which are sustainable over the long run.
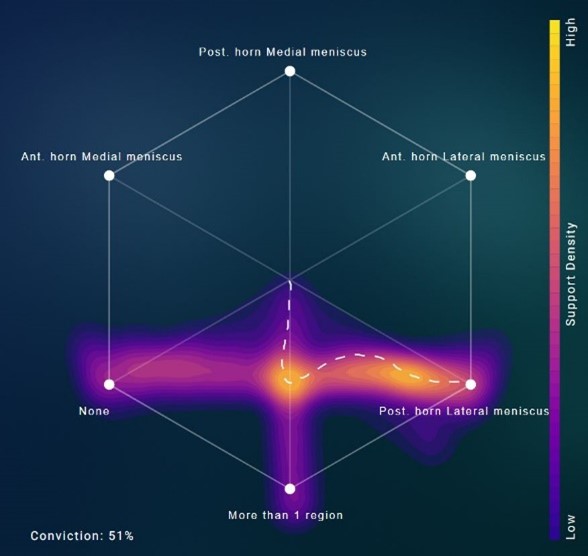
According to Dr. Shah, a key feature of the Swarm platform was in facilitating "anonymized collaborations between participants. This enabled our radiologists to express their true opinions without peer pressure, which is often not the case in collaborative diagnostic settings."
Read the full case study for detailed information.
Authors from UCSF ci2 include Bruno Astuto Arouche Nunes, PhD and Jason Crane, PhD, director of computational core. UCSF Radiology faculty and ci2 members include Kevin McGill, MD, MPH, Thomas Link, MD, PhD, Rina Patel, MD, Sharmila Majumdar, PhD and Valentina Pedoia, PhD. UCSF Radiology clinical fellows - Tyler Gleason, MD and Justin Banaga, MD and residents - Will Fletcher, MD, Kevin Sweetwood, MD and Allen Ye, MD, PhD also played a key role in this study.
Reference
Astuto, B. et al. Automatic Deep Learning Assisted Detection and Grading of Abnormalities in Knee MRI Studies. Radiology: Artificial Intelligence 0, e200165, doi:10.1148/ryai.2021200165.