Breast cancer is the second most common cause of cancer‐related deaths among women in the U.S. Prevention, screening and early detection are critical to decrease mortality rates, particularly in high-risk populations. At this time, existing risk models do not incorporate imaging data and underestimate risk for ethnic minorities.
“Personalized breast cancer risk assessment using imaging biomarkers might help inform decisions regarding more or less frequent screening and preventions strategies,” says Tatiana Kelil, MD, UCSF Center for Intelligent Imaging (ci2) member and UCSF Radiology breast imaging faculty. “Breast MRI contains informative indicators of risk not captured by traditional risk factor assessment models.”
Dr. Kelil received a scholarship from the American Roentgen Ray Society (ARRS) to pursue personalized breast cancer risk assessment using imaging biomarkers and machine learning.
“Background parenchymal enhancement (BPE) is an imaging biomarker which has been shown to be associated with increased breast cancer risk,” says Dr. Kelil. “Machine learning tools can deduce these patterns that might not be discernible to humans from the imaging data and may improve conventional risk prediction models.”
Dr. Kelil has taken the first steps toward the overarching goal of predicting breast cancer risk by focusing on clinical deployment of an automated machine learning pipeline for fibroglandular tissue and BPE quantifications and evaluating their potential as replacement for current workflows based on visual assessment by a radiologist.
Dr. Kelil collaborates closely with Jason Crane, PhD, director of the ci2 Computational Core and Felix Liu, computational and data science researcher on project design, methodology, data acquisition, segmentation, evaluation, deployment and feedback.
“Our current project is laying the foundation for using deep learning for assessment of breast cancer risk in clinical settings, and our project’s primary goal is to significantly improve current risk assessment models by incorporating biomarkers derived from imaging,” says Dr. Kelil.
By working with accurate, quantitatively assessed imaging biomarkers originating from deep learning models, investigators hope that clinicians’ assessments of patient risk will improve which will ultimately lead to an increase in cancer detection rates while unnecessary benign biopsies decrease.
Overall, this work will lay the foundation for using machine learning in the analysis of breast MRI and guide the efficient utilization of breast MRI. In the long term, this could have a major impact in improved screening performance of MRI for women at high risk of breast cancer.
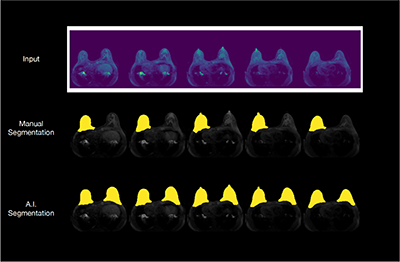