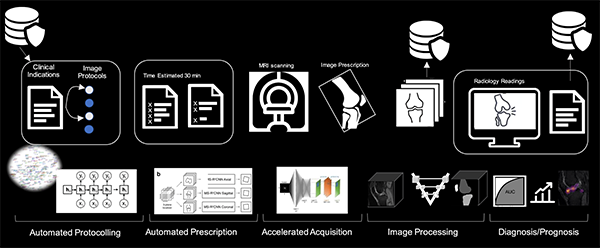
Magnetic resonance imaging (MRI) has become an invaluable tool for osteoarthritis (OA) research based on its three-dimensional nature and soft-tissue contrast. Recent advances related to artificial intelligence (AI) are revolutionizing the use of MRI in clinical research by augmenting activities ranging from image acquisition to post-processing. Applications of deep learning to accelerate MRI acquisition and reconstruction show exciting results. However, fundamental questions remain regarding the most appropriate metrics for evaluating the quality of reconstructed images.
Investigators from the UCSF Center for Intelligent Imaging (ci2) recently reviewed how recent applications of deep learning have improved imaging-based understanding of OA. The work, led by Francesco Calivà, PhD, postdoctoral researcher, and Nikan Namiri, medical student, was recently published in Nature Reviews - Rheumatology.
"Our review discusses the great potential of advancements in deep learning to decrease patient discomfort, by reducing MRI acquisition time through accelerated MRI and super-resolution imaging techniques, which should also result in reducing the costs of patient care," says Nikan Namiri. Overall, deep learning has been used to process large datasets such as from the Osteoarthritis Initiative (OAI) and to analyze knee bone shape, T2 relaxation values and cartilage thickness, which previously required labor-intensive, non-AI approaches. "However, we do see that even with advantages and demonstrated potential of AI-based imaging, there is still a need for clinical validation and quality assurance at this point in time," he continues.
"Improving AI's technological promise can be achieved by increasing the availability of datasets involving large cohorts of participants that include diverse normal and pathological morphologies, as well as multi-institutional collaborations," says Dr. Calivà.
The authors determined that the role of AI in in OA lesion detection is not to provide a final diagnosis but instead rather to serve as an additional input for decision-making. In this context, AI has been tasked with searching for novel image features indicative of short-term and long-term progression of OA to predict disease course on a patient-specific basis.
What's next? According to the authors, future research will likely be devoted to the interpretability and estimation of the uncertainty of deep learning models, with the aim of improving clinician trust in AI for supporting patient care.
Corresponding author on this review is Sharmila Majumdar, PhD. Additional authors from UCSF ci2 include Valentina Pedoia, PhD and Eugene Ozhinsky, PhD. Maureen Dubreuil, MD, a rheumatologist at Boston Medical Center, also contributed.