The National Institutes of Health (NIH) awarded a five-year R01 grant to Valentina Pedoia, PhD, principal investigator and imaging scientist at the UCSF Center for Intelligent Imaging (ci2) for “Ultra-Fast Knee MRI with Deep Learning” to improve the study of joint degeneration, injury and osteoarthritis (OA).
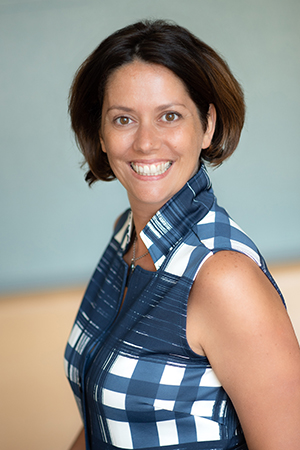
“This is an exciting opportunity as we work to build a novel translational platform to revolutionize knee MR images in research studies,” says Dr. Pedoia. “Our work is also paradigm-shifting in that it may provide a first step towards on real time automatic image inspection and personalized imaging protocolling.”
OA is a widespread and debilitating disease, yet treatment options are limited at this time, and established disease-modifying therapies do not exist. Right now, the solution for treating late-stage knee OA is joint replacement surgery, an invasive option that typically only offers temporary relief. This highlights the importance of preventive efforts and interventions targeting early-stage OA.
Magnetic resonance imaging (MRI) is a valuable tool for studying knee joint degeneration, but it has its limitations including long scanning time, manual post processing and a lack of standardization. Investigators at the UCSF ci2 have recognized that fast, robust, and reliable quantitative knee joint MRI would be a significant step forward in studying joint degeneration, injury, and OA.
Thus far, deep learning has achieved automation of several human tasks including image analysis and interpretation plus revolutionizing the acquisition and reconstruction aspects of the pipeline. Its application to musculoskeletal imaging has showed promising results when applied in a controlled setting, but generalization beyond the statistical distribution of the training set is still an unmet challenge. This is a concern for radiologists because in MRI, this translates into poor performances when trained models are tested on different imaging protocols or images acquired on different MRI systems.
“We plan to leverage recent advances with deep learning and fill these existing gaps,” says Dr. Pedoia. “We’ll study novel integrated models able to simultaneously accelerate MRI acquisition and automate the image processing.”
Typically, fast image acquisition and accurate image post processing are separate problems, but Dr. Pedoia says the neural networks optimization design gives investigators the chance to integrate the two.
“Our study will use both a publicly available benchmark dataset (FastMRI) and an internally collected dataset to build deep learning models that are able to accurately reconstruct under-sampled MRI acquisitions,” continues Dr. Pedoia. “We’ll use a dataset prospectively acquired during this study to validate the clinical applicability of the developed methods.”
The goal is to test the hypothesis that the proposed integrated pipeline can be applied in clinical setting for a fast and intelligent knee scan obtaining image quality comparable to standard acquisition and automated processing accuracy comparable with human reproducibility.
This work will be supported by Dr. Pedoia’s team of co-investigators from the UCSF Center for Intelligent Imaging (ci2) including Peder Larson, PhD, Sharmila Majumdar, PhD, Thomas Link, MD, PhD, Misung Han, PhD and Pablo Damasceno, PhD.
Upon conclusion of this project, the team plans to make annotated image datasets and trained models a shared resource, a centralized, open evaluation platform for MRI reconstruction and image post processing techniques.