As the saying goes, an image is worth a thousand words. Every medical image is replete with countless information about every patient's health – new diagnoses, latent health issues, charting the course of disease, and predicting short- and long-term outcomes. Radiologists are experts at understanding a patient's medical story. They integrate the manifold available sources of information to understand each patient. And with the assistance of new intelligent analytics, especially those that utilize artificial intelligence and machine learning, imaging contains an even greater wealth of information that can be used to optimize and precisely tailor high-quality care for a broader and more diverse group of patients. Artificial intelligence magnifies the impact of imaging.
The Center for Intelligent Imaging (ci2) was launched in 2018 in the UCSF Department of Radiology and Biomedical Imaging and focuses on using new techniques to enhance image acquisition, improve image quality, enable quantitative analysis of images and extract new information from images. Ci2 brings together imaging experts across an array of clinical and scientific backgrounds, from radiologists, surgeons, cardiologists and oncologists to engineers, informatics experts and students, to create an ecosystem for the study of how best develop and apply new artificial intelligence technology and intelligent analytic techniques for imaging. Imaging is a window into biology, physiology and disease. The construction of images from biological data is complex. Utilizing AI and machine learning, ci2 teams are working to overcome the limitations of traditional data acquisition and analysis in imaging. New technology, especially in artificial intelligence, has enabled faster, more robust and higher-quality imaging. It has also launched the ability to extract quantitative data from images.
Ci2 is developing technology that optimizes patient health. Some of the recent successes of the teams within ci2 are highlighted below.
Clinical Deployment
The ci2 Clinical Deployment team has developed a framework for evaluating whether an AI algorithm should be deployed for routine clinical usage, and if so, how. The framework evaluates the balance between the benefits provided by the algorithm and the costs and risks incurred by deployment. On the benefit side, the framework considers the breadth and depth of the potential improvement, the effect on workload and efficiency, and compares this to the current workflow or standard of care. On the cost side, likelihood of erroneous outputs, detectability and correctability of those errors, and patient impact of uncorrected errors are evaluated. The financial costs of deployment, maintenance and training are considered, and potential unintended consequences are explored, including creation or exacerbation of health care disparities, delays in care and adverse effects on radiologist performance.
For algorithms where the balance of these factors is favorable for deployment, the form of integration with the clinical environment and workflow is designed. This takes into consideration how the AI results should be presented, how they should be contextualized and how they should be stored as part of the medical record. A post-deployment monitoring plan is developed.
This framework has been successfully employed to guide the deployment of the BunkerHill Coronary Artery Calcification scoring algorithm and will soon be applied to several ci2-developed algorithms, including brain tumor volumetrics.
Extramural Funding
In April 2021, researchers in the ci received a five-year R01 grant from the National Institutes of Health for "UltraFast Knee MRI with Deep Learning" to improve the study of joint degeneration, injury and osteoarthritis. This study is paradigm-shifting in that it may provide a first step toward the integration of accelerated image acquisition, fully automatic image inspection and personalized imaging protocoling. The integration of ultrafast MRI image acquisition, online image reconstruction and post-processing opens new horizons of opportunities and different use-cases to facilitate the translation of fast MRI techniques.
The integrated pipeline the investigators are developing has the potential to characterize patients automatically based on quantitative features at the time of data acquisition and potentially to modify the MRI protocol dynamically by adding sequences to better tailor them to patient needs using real-time precision imaging. An ultrafast MRI-based preliminary patient triage system can open new possibilities on the use of accelerated MRI. If ultrafast MRI is used for online patient triage and personalization of image protocoling, sequences that are optimally acquired and optimized based on patient characteristics would be available at the time of study interpretation by the radiologist. This would help the radiologist provide a better and more precise assessment of abnormalities. Real-time automatic image processing and interpretation of highly accelerated MRI acquisition may change drastically the musculoskeletal radiology scenario.
Federated Learning, AI and Clinical Deployment at UCSF – Lessons Learned from COVID-19 Modeling
Early in the pandemic, predicting oxygen requirements for incoming patients was important, given the limited availability of respirators and hospital resources. At that time, no single hospital had sufficient data to train a predictive AI model capable of making such predictions. It was clear that the only short-term option would require pooling data collaboratively among multiple sites. However, directly sharing medical data is challenging.
This led a team at ci2, to turn its attention to federated learning, a privacy-preserving method capable of training neural networks on decentralized data that enables sensitive patient information to remain securely at each institution. The team included Jae Ho Sohn, MD, assistant professor, Pablo Damasceno, PhD, senior data scientist, Peter Storey, manager of scientific computing services, Jed Chan, systems administrator and Jeff Block, director of infrastructure, along with Wyatt Tellis, PhD, director of innovation and analytics, among others.
UCSF collaborated with 20 sites worldwide using federated learning to develop a model for predicting COVID-19 outcomes from chest X-rays, labs and vitals collected during an emergency room visit. Remarkably, the modeling was completed in 5 months and achieved an average area under the curve (AUC) >0.92 for predicting outcomes at 24 and 72hours from time of presentation (Nature Medicine; 27, pages 1735–1743. 2021). The project demonstrated the power of AI methodologies combined with federated learning to collaboratively develop robust solutions to critical public health problems in a timely fashion. The work has since paved the way for a multi-center effort focused on predictive modeling in prostate cancer (PI: Peder Larson, PhD, UCSF/UCLA).
Translating such methods into the clinic safely and efficiently is a key goal. This requires the development of frameworks capable of deploying clinically integrated models, work being led by Carolina Ramirez and James Hawkins (data scientists, ci2 Computational Core) in collaboration with UCSF's Apex Enabled Research (AER link) and under the oversight of ci2's Clinical Deployment pillar team, led by Dr. Mongan and Jason Crane, PhD.
This article is reposted from the recent issue of Images from the UC San Francisco Department of Radiology and Biomedical Imaging. Read more articles from the publication here.
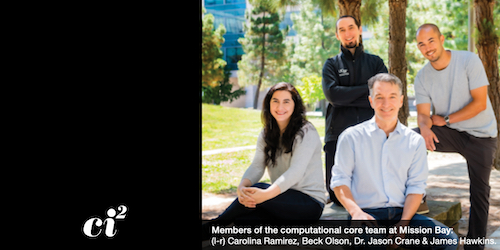